A Neural Network Text Classification Method Fused with Multiple Knowledge Graphs
A knowledge map and neural network technology, applied in the fields of natural language processing and data mining, can solve problems such as inaccessible coverage, impact modeling, noise, etc., achieve reliable, accurate and robust classification, and improve understanding
- Summary
- Abstract
- Description
- Claims
- Application Information
AI Technical Summary
Problems solved by technology
Method used
Image
Examples
Embodiment Construction
[0054] The present invention will be further described in detail below in conjunction with the accompanying drawings and specific embodiments.
[0055] figure 1 It is an implementation flowchart of a neural network text classification method fused with multi-knowledge graphs in the present invention. Such as figure 1 As shown, the method includes the following steps:
[0056] Step A: Input the text in the training set into the long short-term memory network to obtain the context vector of the text. Specifically include the following steps:
[0057] Step A1: For any text D, perform word segmentation processing, and use the word embedding tool to convert the words in the text into word vector form. The calculation formula is as follows:
[0058] v=W·v'
[0059] Among them, each word in the text is randomly initialized as a d'-dimensional real number vector v'; W is the word embedding matrix, W∈R d ×d′ , which is obtained from a large-scale corpus trained in a neural netwo...
PUM
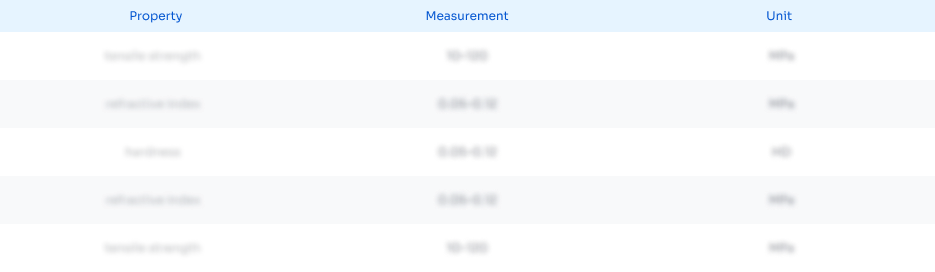
Abstract
Description
Claims
Application Information
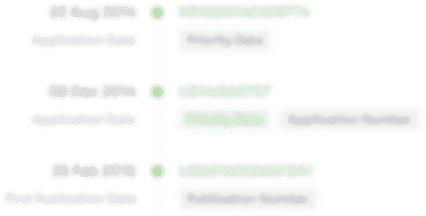
- R&D Engineer
- R&D Manager
- IP Professional
- Industry Leading Data Capabilities
- Powerful AI technology
- Patent DNA Extraction
Browse by: Latest US Patents, China's latest patents, Technical Efficacy Thesaurus, Application Domain, Technology Topic.
© 2024 PatSnap. All rights reserved.Legal|Privacy policy|Modern Slavery Act Transparency Statement|Sitemap