Fine-grained image classification method based on depth convolution neural network
A deep convolution and neural network technology, applied in the field of fine-grained image classification based on deep convolutional neural network, can solve problems such as slow convergence speed, high computational complexity, and dependence on accurate component detection
- Summary
- Abstract
- Description
- Claims
- Application Information
AI Technical Summary
Problems solved by technology
Method used
Image
Examples
Embodiment Construction
[0063] In view of the main challenges of fine-grained image classification (similarity between classes and diversity within classes), this invention proposes to improve the performance of deep convolutional neural networks by using class label hierarchical structure relations, cascading softmax loss and generalization large-margin loss. Fine-grained image classification performance. Specifically, the present invention improves the fine-grained image classification accuracy of the deep convolutional neural network from the following two aspects. First, for a given deep convolutional neural network, in order to better utilize the h-level hierarchical structure relationship between fine-grained class labels, the present invention proposes to use h fully connected layers to replace the last fully connected neural network Layers, the parameters of these new fully connected layers are learned with the cascaded softmax loss proposed by the present invention. Second, the present inve...
PUM
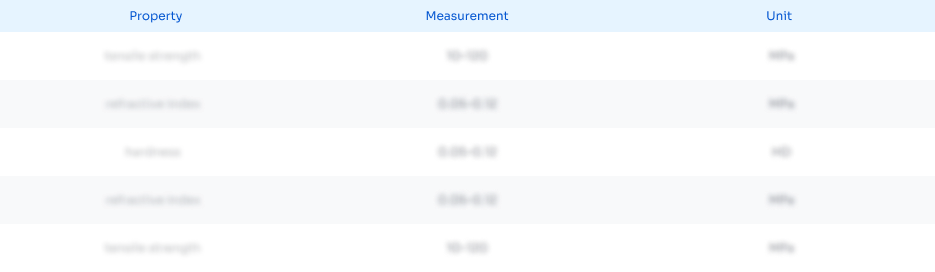
Abstract
Description
Claims
Application Information
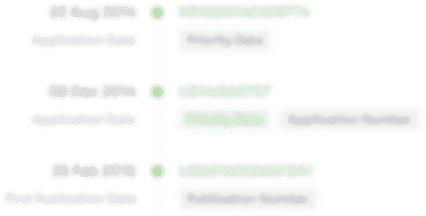
- R&D Engineer
- R&D Manager
- IP Professional
- Industry Leading Data Capabilities
- Powerful AI technology
- Patent DNA Extraction
Browse by: Latest US Patents, China's latest patents, Technical Efficacy Thesaurus, Application Domain, Technology Topic, Popular Technical Reports.
© 2024 PatSnap. All rights reserved.Legal|Privacy policy|Modern Slavery Act Transparency Statement|Sitemap|About US| Contact US: help@patsnap.com