Low rank image denoising method
An image and image matrix technology, applied in the field of image denoising, can solve the problems affecting the accuracy, sparseness, and unstable solutions of image restoration, and achieve the effects of improving accuracy, enhancing stability, and avoiding too sparse
- Summary
- Abstract
- Description
- Claims
- Application Information
AI Technical Summary
Problems solved by technology
Method used
Image
Examples
Embodiment 1
[0060] Such as figure 1 with image 3 As shown, the present invention provides a method for denoising a low-rank image, comprising the steps of:
[0061] 1) Input an image D containing noise;
[0062] 2) Firstly, the matrix D∈R to be restored m×n Perform singular value decomposition Get his left singular vector U=(u 1 , u 2 ,...,u m )∈R m×m , right singular vector V=(v 1 ,v 2 ,...,v m )∈R n×n and the singular value Σ r =diag(σ 1 ,σ 2 ,...,σ r );
[0063] 3) According to the definition of truncated nuclear norm Arrange the singular values from small to large, remove the largest r-t singular values, and take the left singular vector F=(u 1 , u 2 ,...,u t ) T and right singular vector G=(v 1 ,v 2 ,...,v t ) T ;
[0064] 4) According to the objective function and constraints, an image denoising model based on truncated kernel norm and Frobenius norm is established,
[0065]
[0066] Where A represents the original image; E represents noise, ||E|| ...
Embodiment 2
[0099] Such as figure 2 Shown, (a) is original image, and (b) is the noise-containing image of original image, utilizes NNR, NNF, TNNR and the method for embodiment 1 to carry out denoising process to noise-containing image (b) respectively, four kinds of methods The PSNRs are 25.3, 26.1, 27.5, and 28.4 respectively, and the denoised images (c), (d), (e) and (f) are obtained respectively. According to the comparison, the image (f) obtained by the method of Example 1 The closest to the original image (a), the best denoising effect.
PUM
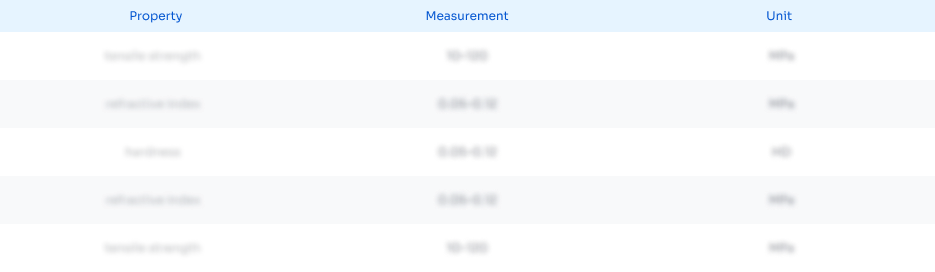
Abstract
Description
Claims
Application Information
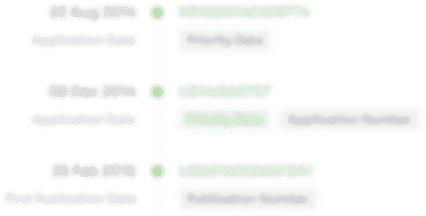
- R&D Engineer
- R&D Manager
- IP Professional
- Industry Leading Data Capabilities
- Powerful AI technology
- Patent DNA Extraction
Browse by: Latest US Patents, China's latest patents, Technical Efficacy Thesaurus, Application Domain, Technology Topic, Popular Technical Reports.
© 2024 PatSnap. All rights reserved.Legal|Privacy policy|Modern Slavery Act Transparency Statement|Sitemap|About US| Contact US: help@patsnap.com