Deep-learning-based method for intermittent process fault detection
A technology of fault detection and deep learning, applied in electrical testing/monitoring, testing/monitoring control systems, instruments, etc., can solve problems such as slow modeling speed, slow fault detection speed, complex modeling data, etc., to improve detection accuracy efficiency, rapid modeling and detection
- Summary
- Abstract
- Description
- Claims
- Application Information
AI Technical Summary
Problems solved by technology
Method used
Image
Examples
Embodiment Construction
[0036] Assuming that the total number of batches of a batch of equal-length data X is I, the number of samples in each batch is J, and the number of variables is K, then the method of batch expansion is used. Such as figure 1 As shown, the three-dimensional data (J×K×I) is expanded into a two-dimensional matrix (JK×I) in the batch direction. Among them, each column of the expanded matrix is a batch of data, and finally the training data is obtained: X={x 1 ,x 2 ,...,x I}∈R JK×I .
[0037] Unlike Principal Component Analysis (PCA), the self-encoding network uses a nonlinear activation function to perform nonlinear transformations, such as nonlinear functions such as sigmoid function or tanh function, in order to enable the self-encoding network to extract features and reconstruct data, the original data needs to be scaled, otherwise the autoencoder network will not be able to reconstruct the data in a non-linear manner. Take the tanh activation function as an example: ...
PUM
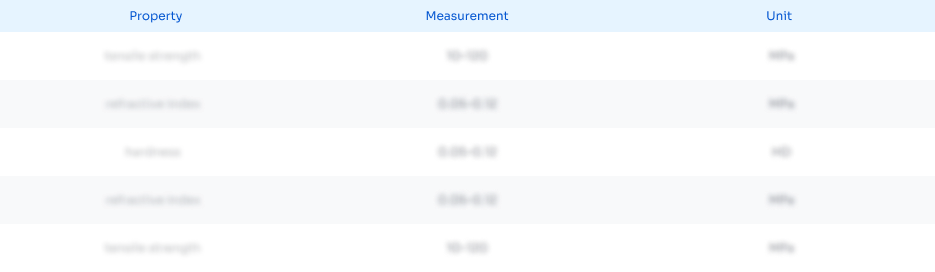
Abstract
Description
Claims
Application Information
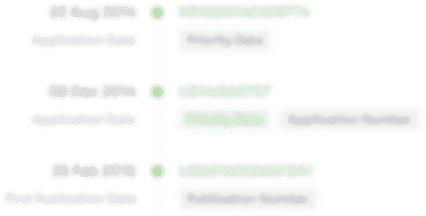
- R&D Engineer
- R&D Manager
- IP Professional
- Industry Leading Data Capabilities
- Powerful AI technology
- Patent DNA Extraction
Browse by: Latest US Patents, China's latest patents, Technical Efficacy Thesaurus, Application Domain, Technology Topic.
© 2024 PatSnap. All rights reserved.Legal|Privacy policy|Modern Slavery Act Transparency Statement|Sitemap