Personalized traffic accident risk prediction and recommendation method based on depth learning
A traffic accident and risk prediction technology, applied in the field of deep learning, can solve problems such as weak correlation, lack of consideration of traffic flow correlation, and less traffic accident prediction, so as to achieve the effect of improving accuracy
- Summary
- Abstract
- Description
- Claims
- Application Information
AI Technical Summary
Problems solved by technology
Method used
Image
Examples
Embodiment
[0044] see figure 1 with figure 2 , the invention discloses a personalized traffic accident risk prediction and recommendation method based on deep learning, comprising the following steps:
[0045] S1. According to the distribution of urban roads, the city is divided into I×J grid areas (reference image 3 shown).
[0046] S2. According to the historical traffic accident data, calculate the grid coordinates corresponding to each traffic accident and the traffic accident data in each grid area and time period. Step S2 specifically includes:
[0047] S21. Extract the key fields {ID, E, τ, X, Y} in the historical traffic accident data, wherein ID represents the traffic accident number, E represents the description of the traffic accident, τ represents the alarm time, and X represents the longitude of the location of the traffic accident , Y represents the latitude of the location of the traffic accident.
[0048] S22. Preprocessing the historical traffic accident data to d...
PUM
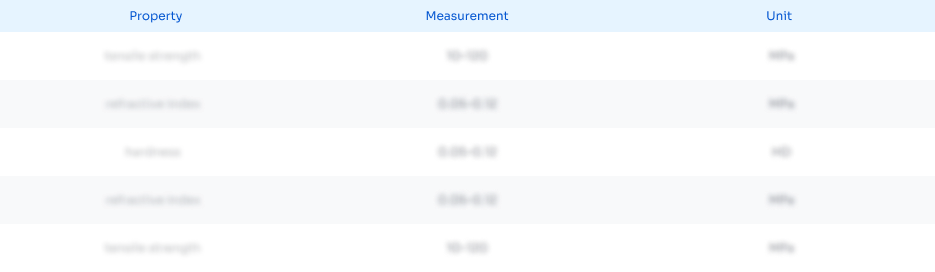
Abstract
Description
Claims
Application Information
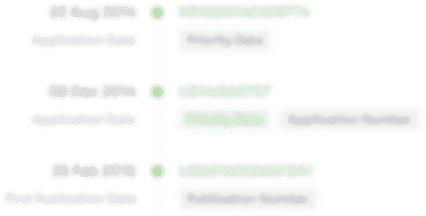
- R&D Engineer
- R&D Manager
- IP Professional
- Industry Leading Data Capabilities
- Powerful AI technology
- Patent DNA Extraction
Browse by: Latest US Patents, China's latest patents, Technical Efficacy Thesaurus, Application Domain, Technology Topic.
© 2024 PatSnap. All rights reserved.Legal|Privacy policy|Modern Slavery Act Transparency Statement|Sitemap