Bearing fault diagnosis method based on EEMD-AR model and DBN
A fault diagnosis and AR model technology, applied in the field of wind power, can solve problems such as nonlinearity, difficulty in fault feature extraction, non-stationary fault signals, etc., and achieve the effect of high accuracy and high precision fault diagnosis
- Summary
- Abstract
- Description
- Claims
- Application Information
AI Technical Summary
Problems solved by technology
Method used
Image
Examples
Embodiment Construction
[0016] The present invention will be further described below in conjunction with specific drawings and embodiments.
[0017] The present invention proposes a wind turbine bearing fault diagnosis method based on the EEMD-AR model and DBN. Due to the nonlinear change of the bearing vibration signal, it is first decomposed into multiple IMF components through the aggregation empirical mode decomposition (EEMD) method, and the selected For the first four IMF components, an autoregressive (referred to as AR) model is established, and the autoregressive coefficient and corresponding variance are calculated for each AR model as the input parameters of the deep belief network (referred to as the DBN network); extracted after EEMD and AR preprocessing The low-order features of the signal are obtained; and then the powerful feature layered extraction and generalization capabilities of the DBN network are used to mine representative high-order features from the input parameters for fault ...
PUM
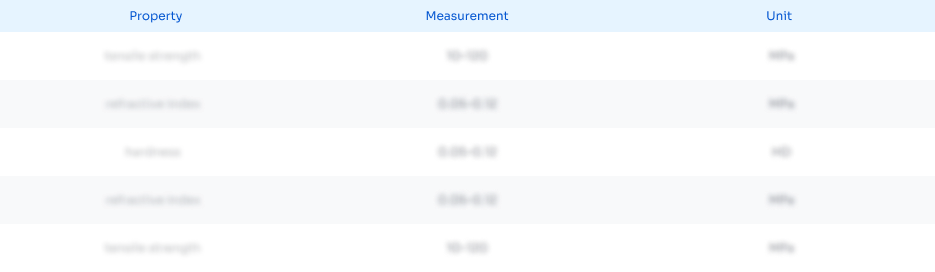
Abstract
Description
Claims
Application Information
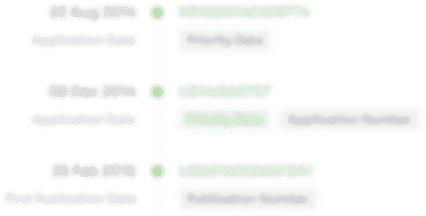
- R&D
- Intellectual Property
- Life Sciences
- Materials
- Tech Scout
- Unparalleled Data Quality
- Higher Quality Content
- 60% Fewer Hallucinations
Browse by: Latest US Patents, China's latest patents, Technical Efficacy Thesaurus, Application Domain, Technology Topic, Popular Technical Reports.
© 2025 PatSnap. All rights reserved.Legal|Privacy policy|Modern Slavery Act Transparency Statement|Sitemap|About US| Contact US: help@patsnap.com