Environment Adaptive Import Method for Intelligent Driving Vehicles in Urban Environment
A technology of intelligent driving and vehicle environment, applied in the direction of adaptive control, instrumentation, control/adjustment system, etc., can solve the problem of inability to simulate automatic integration into driving experience, and achieve the effect of improving adaptability
- Summary
- Abstract
- Description
- Claims
- Application Information
AI Technical Summary
Problems solved by technology
Method used
Image
Examples
Embodiment
[0049] The invention considers the selection of the target gap and the timing of expected import, and proposes it based on the least square strategy iteration algorithm.
[0050] In this method, the leading vehicle, the following vehicle and the merging vehicle in a candidate gap are regarded as units and merged into the system for reinforcement learning modeling. In the process of policy optimization, the maximum action value functions of all candidate gaps are compared, and the policy corresponding to the maximum value is selected as the output policy. In the process of unit system reinforcement learning modeling, the evaluation indicators such as safety, comfort and timeliness are comprehensively considered, and the current weighted comprehensive reward value model is established. At the same time, the action space setting includes two-dimensional variables, namely the longitudinal speed decision variable and The lateral speed decision variable decouples the lateral and lon...
Embodiment 2
[0088] The specific process of optimization training of the environment adaptive import strategy method based on LSPI algorithm is as follows:
[0089] (1) Initialization strategy π 0 and sample set D 0 ;
[0090] (2) Run Vissim+PreScan joint traffic simulation platform;
[0091] (3) Obtain the imported environment information from the simulation environment, and extract the state vector s t ;
[0092] (4) Calculate the action variable a according to the greedy strategy t ; If a random action is taken, the import gap and import action will be selected with uniform probability; if the action is selected by an agent, then the maximum value function of all candidate gaps will be compared, and the gap and action corresponding to the maximum value will be selected, and returned as the target sink into gaps and agents into actions. The simulation platform executes the import action a t , and update the import scene.
[0093] (5) The unmanned vehicle perceives the state vecto...
PUM
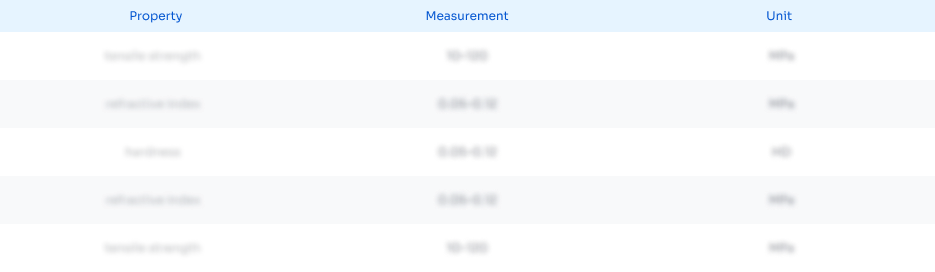
Abstract
Description
Claims
Application Information
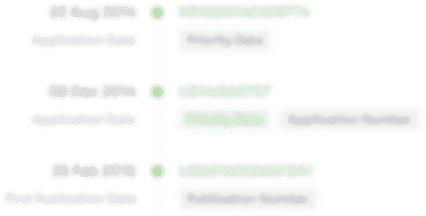
- R&D Engineer
- R&D Manager
- IP Professional
- Industry Leading Data Capabilities
- Powerful AI technology
- Patent DNA Extraction
Browse by: Latest US Patents, China's latest patents, Technical Efficacy Thesaurus, Application Domain, Technology Topic, Popular Technical Reports.
© 2024 PatSnap. All rights reserved.Legal|Privacy policy|Modern Slavery Act Transparency Statement|Sitemap|About US| Contact US: help@patsnap.com