A method for generating a new sample base on a generative adversarial network and an adaptive proportion
An adaptive, new sample technology, applied in the field of deep neural network, can solve the problem of model convergence decline, affecting the performance of gradient update algorithm, etc.
- Summary
- Abstract
- Description
- Claims
- Application Information
AI Technical Summary
Problems solved by technology
Method used
Image
Examples
Embodiment Construction
[0074] The technical solution of the present invention will be further described and illustrated through specific embodiments below, so that the technical solution will be clearer and clearer.
[0075] The present invention decouples the noise from the gradient update algorithm, and proposes a method for generating new samples based on the generative confrontation network and adaptive ratio, directly adding the noise generated by the generative confrontation network and the distribution of the original sample to the input sample (original sample) , to get a new sample, the new sample adjusts the proportion of the noise and the original sample according to the adaptive ratio, when the DNN loss function is steep, increase the original sample ratio, reduce the noise ratio, and avoid the DNN from crossing the minimum value; when the DNN loss function is flat, reduce Smaller original sample ratio and larger noise ratio are beneficial to speed up the convergence speed of DNN. The in...
PUM
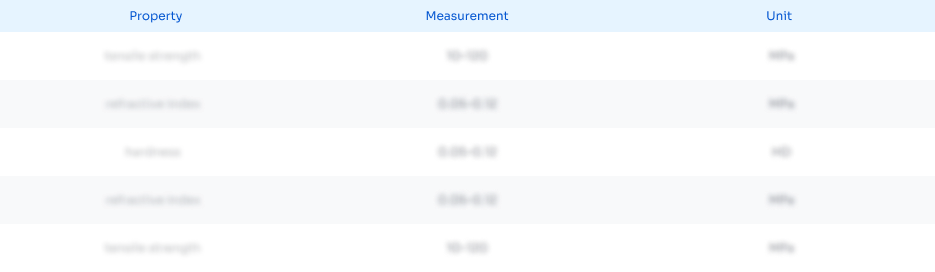
Abstract
Description
Claims
Application Information
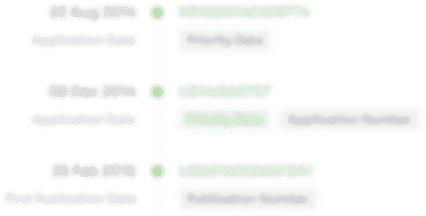
- R&D Engineer
- R&D Manager
- IP Professional
- Industry Leading Data Capabilities
- Powerful AI technology
- Patent DNA Extraction
Browse by: Latest US Patents, China's latest patents, Technical Efficacy Thesaurus, Application Domain, Technology Topic.
© 2024 PatSnap. All rights reserved.Legal|Privacy policy|Modern Slavery Act Transparency Statement|Sitemap