A domain adaptive image semantic segmentation method based on antagonistic learning
A technology of semantic segmentation and domain self-adaptation, which is applied in the fields of instruments, biological neural network models, character and pattern recognition, etc., and can solve the problems of high-dimensional feature complexity and low-dimensional features that cannot be well adapted
- Summary
- Abstract
- Description
- Claims
- Application Information
AI Technical Summary
Problems solved by technology
Method used
Image
Examples
Embodiment Construction
[0030] It should be noted that, in the case of no conflict, the embodiments in the present application and the features in the embodiments can be combined with each other. The present invention will be further described in detail below in conjunction with the drawings and specific embodiments.
[0031] figure 1 It is a system architecture diagram of a domain-adaptive image semantic segmentation method based on adversarial learning in the present invention. It mainly includes domain adaptation, network structure, and output space adaptation.
[0032] The domain-adaptive image semantic segmentation method first inputs the source domain and target domain images, and passes them to the segmentation network to predict the segmentation output of the source domain and the target domain; the source prediction obtained from the source output generates the segmentation loss of the source domain; then the output is used as The input of the discriminator network generates an adversarial ...
PUM
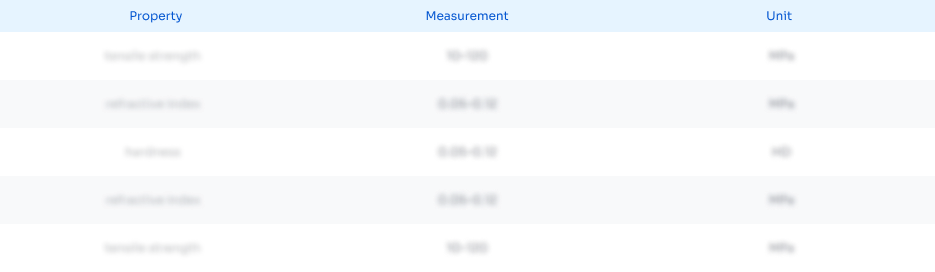
Abstract
Description
Claims
Application Information
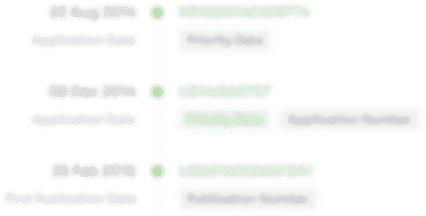
- R&D Engineer
- R&D Manager
- IP Professional
- Industry Leading Data Capabilities
- Powerful AI technology
- Patent DNA Extraction
Browse by: Latest US Patents, China's latest patents, Technical Efficacy Thesaurus, Application Domain, Technology Topic.
© 2024 PatSnap. All rights reserved.Legal|Privacy policy|Modern Slavery Act Transparency Statement|Sitemap