A Method for Reducing Energy Consumption in Large-Scale Distributed Machine Learning Systems
A machine learning and distributed technology, applied in instrumentation, resource allocation, energy-saving computing, etc., can solve the problems of server power waste, overall performance degradation, and long iteration time, so as to reduce system energy consumption, improve utilization, and shorten execution time. effect of time
- Summary
- Abstract
- Description
- Claims
- Application Information
AI Technical Summary
Problems solved by technology
Method used
Image
Examples
Embodiment Construction
[0020] The method for reducing the energy consumption of large-scale distributed machine learning proposed by the present invention has the following steps:
[0021] Step 1: The scheduler collects the real-time information of the CPU, GPU, memory, and disk I / O of the working machine and sends it to the state memory.
[0022] Step 2: The state memory uses the received real-time information of the processor, memory, and disk I / O to calculate the load status of the work machine (CPU usage, GPU usage, memory usage, and disk I / O usage).
[0023] Step 3: The scheduling policy manager reads the load information on the state memory. The load status of different working machines at the same time is used to predict the load type of machine learning tasks (computing-intensive, I / O-intensive, GPU-accelerated, hybrid), and the load curves at different times are used to predict the working machines in the future load.
[0024] Step 4: When the machine learning task arrives, first use the ...
PUM
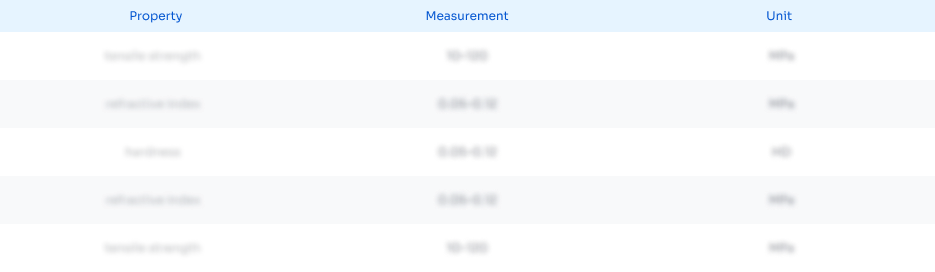
Abstract
Description
Claims
Application Information
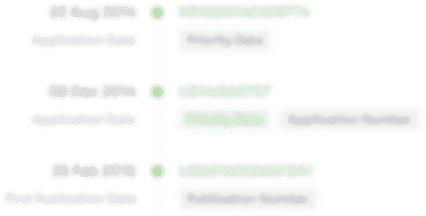
- R&D Engineer
- R&D Manager
- IP Professional
- Industry Leading Data Capabilities
- Powerful AI technology
- Patent DNA Extraction
Browse by: Latest US Patents, China's latest patents, Technical Efficacy Thesaurus, Application Domain, Technology Topic, Popular Technical Reports.
© 2024 PatSnap. All rights reserved.Legal|Privacy policy|Modern Slavery Act Transparency Statement|Sitemap|About US| Contact US: help@patsnap.com