A method and system for cross-modal hash retrieval fusing supervisory information
A cross-modal, hashing technology, applied in the field of cross-modal hash retrieval method and system that fuses supervisory information, can solve the problems of not being able to fully mine the complex relationship between multi-modal data, and achieve similarity and semantics Consistency, the effect of reducing quantization error
- Summary
- Abstract
- Description
- Claims
- Application Information
AI Technical Summary
Problems solved by technology
Method used
Image
Examples
Embodiment 1
[0049] This embodiment discloses a cross-modal hash retrieval method that fuses supervision information, such as Figure 1-2 shown, including the following steps:
[0050] Phase 1: Unified Hash Code Learning
[0051] Step 1: Build three networks: image network, text network and fusion network. (1) The CNN-F network used by the image network. The original CNN-F model has a total of 8 layers, including 5 convolutional layers and 3 fully connected layers. (2) For the text modality, first represent each text sample as a bag-of-word (BOW) vector, and then input the BOW vector to a text network with two fully connected layers. In particular, the number of hidden units in the last layer of image and text networks is equal, and different values are set according to different encoding lengths and data sets. (3) The fusion network consists of two fully connected layers, which combine the outputs of the image and text networks pairwise. In order to obtain a unified hash code, the ...
Embodiment 2
[0127] The purpose of this embodiment is to provide a computing device.
[0128] A computer system, comprising a memory, a processor, and a computer program stored in the memory and operable on the processor, when the processor executes the program, it realizes:
[0129] Construct image network, text network and fusion network;
[0130] Obtain image and text feature training sample pairs, input image network and text network respectively;
[0131] Using the output features of the image network and the text network as the input of the fusion network, and defining the output of the fusion network;
[0132] Constructing an objective function for learning a unified hash code according to the output of the fusion network and the similarity between pairs;
[0133] Solving the objective function to obtain a unified hash code;
[0134] The unified hash code is used as supervisory information, combined with semantic information, to train a modality-specific hash network.
Embodiment 3
[0136] The purpose of this embodiment is to provide a computer-readable storage medium.
[0137] A computer-readable storage medium, on which a computer program is stored, and when the program is executed by a processor, the following steps are implemented:
[0138] Construct image network, text network and fusion network;
[0139] Obtain image and text feature training sample pairs, input image network and text network respectively;
[0140] Using the output features of the image network and the text network as the input of the fusion network, and defining the output of the fusion network;
[0141] Constructing an objective function for learning a unified hash code according to the output of the fusion network and the similarity between pairs;
[0142] Solving the objective function to obtain a unified hash code;
[0143] The unified hash code is used as supervisory information, combined with semantic information, to train a modality-specific hash network.
PUM
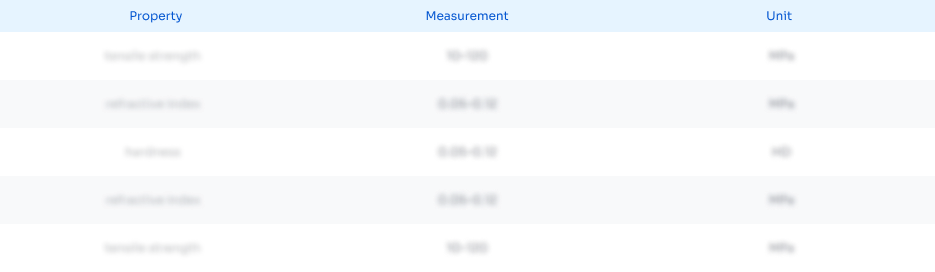
Abstract
Description
Claims
Application Information
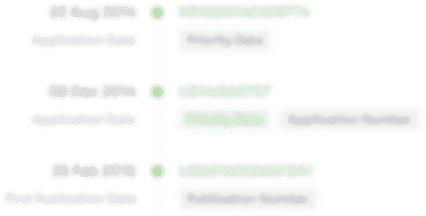
- R&D
- Intellectual Property
- Life Sciences
- Materials
- Tech Scout
- Unparalleled Data Quality
- Higher Quality Content
- 60% Fewer Hallucinations
Browse by: Latest US Patents, China's latest patents, Technical Efficacy Thesaurus, Application Domain, Technology Topic, Popular Technical Reports.
© 2025 PatSnap. All rights reserved.Legal|Privacy policy|Modern Slavery Act Transparency Statement|Sitemap|About US| Contact US: help@patsnap.com