Conversational recommendation method and system based on graph neural network and review similarity
A recommendation method and neural network technology, applied in the fields of artificial intelligence and recommendation systems, can solve problems such as the inability to accurately capture the complex dependencies of commodities in the session, the inability to accurately model user preferences, and the difficulty in obtaining recommendation effects, etc., to increase reliability Effects of interpretability, optimized weights, and improved accuracy
- Summary
- Abstract
- Description
- Claims
- Application Information
AI Technical Summary
Problems solved by technology
Method used
Image
Examples
Embodiment 1
[0103] The overall model framework of the present invention is as follows figure 2 shown. Depend on figure 2 It can be seen that the main frame structure of the present invention includes a global graph module, a local graph module, a session generation module and a candidate product prediction module based on the similarity of reviews. Among them, the global graph module based on comment similarity first uses the idea of graph attention network to generate attention weights according to the importance of each connection; the global graph based on comment similarity is the global graph neighbor information obtained after preprocessing and adjacent edge weights; after the global graph is constructed, the nodes in the graph, that is, the commodities in the session, are updated and learned by means of a graph attention neural network, and the final weighted summation is used to obtain the global graph commodity representation. The local graph module uses the paired commodit...
Embodiment 2
[0105] The specific steps of the conversation recommendation method based on graph neural network and comment similarity of the present invention are as follows:
[0106] S1. Session sequences required for establishing a training set and a test set: First, it is necessary to obtain user interaction records on an e-commerce website, and then perform preprocessing operations on them to obtain session sequences that meet the training requirements. The specific steps are as follows:
[0107] S101. Download a data set of an e-commerce website that has been published on the Internet, and use it as raw data for constructing a session sequence.
[0108] For example: There are many publicly available historical datasets of user behavior for recommender systems, such as Amazon's Pet Supplies dataset.
[0109] The data format in the Pet Supplies dataset is as follows:
[0110]
[0111] Where reviewerID is the user ID; asin is the product ID; reviewerName is the user's nickname; review...
Embodiment 3
[0240] Based on the conversational recommendation system based on graph neural network and comment similarity according to Embodiment 2, the system includes:
[0241] The session sequence construction unit of the training set and the test set first needs to obtain the user's interaction records on the e-commerce website, and then preprocess them to obtain the session sequence that meets the training requirements; the session sequence construction unit of the training set and the test set include,
[0242] The original data acquisition unit is responsible for downloading the data sets of e-commerce websites that have been published on the Internet as the original data for constructing the session sequence;
[0243] The original data preprocessing unit is responsible for setting the time span of the session to a certain time period, and constructing the training set and test set session sequence that meets the conditions, so as to construct the session sequence of the training s...
PUM
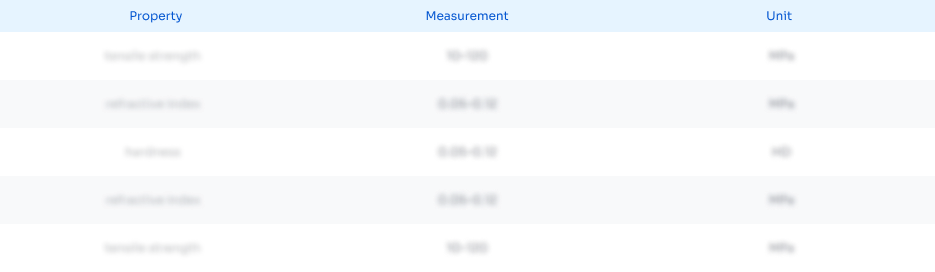
Abstract
Description
Claims
Application Information
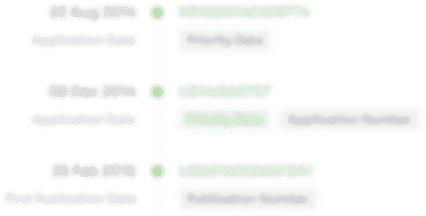
- R&D Engineer
- R&D Manager
- IP Professional
- Industry Leading Data Capabilities
- Powerful AI technology
- Patent DNA Extraction
Browse by: Latest US Patents, China's latest patents, Technical Efficacy Thesaurus, Application Domain, Technology Topic, Popular Technical Reports.
© 2024 PatSnap. All rights reserved.Legal|Privacy policy|Modern Slavery Act Transparency Statement|Sitemap|About US| Contact US: help@patsnap.com