A binocular depth estimation method based on depth neural network
A deep neural network and depth estimation technology, applied in the field of multimedia image processing, can solve problems such as inaccurate depth values, difficult calibration of hardware equipment, and sparse depth information, so as to reduce information loss, ensure accuracy, improve accuracy and robustness. sticky effect
- Summary
- Abstract
- Description
- Claims
- Application Information
AI Technical Summary
Problems solved by technology
Method used
Image
Examples
Embodiment Construction
[0036] The present invention will be further described in detail below in conjunction with the accompanying drawings and through specific embodiments. The following embodiments are only descriptive, not restrictive, and cannot limit the protection scope of the present invention.
[0037] 1) Carry out corresponding image preprocessing such as cutting and transforming the input left and right viewpoint images for data enhancement.
[0038]The present invention adopts images of left and right angles of view acquired by a binocular camera as network input, and can output a monocular depth map in a left camera coordinate system or a right camera coordinate system. For the convenience of description, the output monocular depth map mentioned in this article is the depth map of the left picture. The input image in the present invention requires RGB images of left and right perspectives, so part of the data in the artificially synthesized dataset SceneFlow and the KITTI2015 dataset in ...
PUM
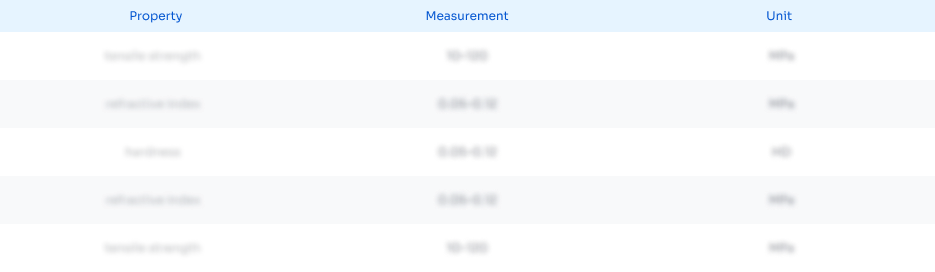
Abstract
Description
Claims
Application Information
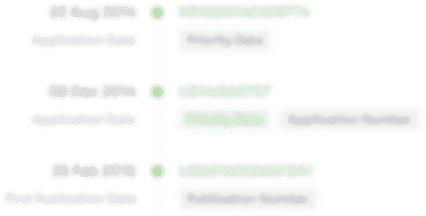
- R&D Engineer
- R&D Manager
- IP Professional
- Industry Leading Data Capabilities
- Powerful AI technology
- Patent DNA Extraction
Browse by: Latest US Patents, China's latest patents, Technical Efficacy Thesaurus, Application Domain, Technology Topic, Popular Technical Reports.
© 2024 PatSnap. All rights reserved.Legal|Privacy policy|Modern Slavery Act Transparency Statement|Sitemap|About US| Contact US: help@patsnap.com