A K-nearest neighbor and multi-class merge based density peak clustering method and image segmentation system
A density peak and clustering method technology, applied in the field of graphic recognition, can solve the problems of easy error propagation, low clustering quality, and inconsistent local density measurement methods when assigning remaining points
- Summary
- Abstract
- Description
- Claims
- Application Information
AI Technical Summary
Problems solved by technology
Method used
Image
Examples
Embodiment Construction
[0077] In order to make the object, technical solution and advantages of the present invention more clear, the present invention will be further described in detail below in conjunction with the examples. It should be understood that the specific embodiments described here are only used to explain the present invention, not to limit the present invention.
[0078] The present invention is based on the density peak clustering algorithm (KM-DPC) of K-nearest neighbor and multi-category merging, uses the defined density calculation method to describe the sample distribution, adopts a new evaluation index to obtain the clustering center; then combines the idea of K-nearest neighbor to design iterative distribution The strategy classifies the remaining points accurately; a local class merging method is given to prevent splitting of classes containing multiple density peak points. Simulation results show that KM-DPC significantly outperforms DPC on 22 different datasets.
[0079]...
PUM
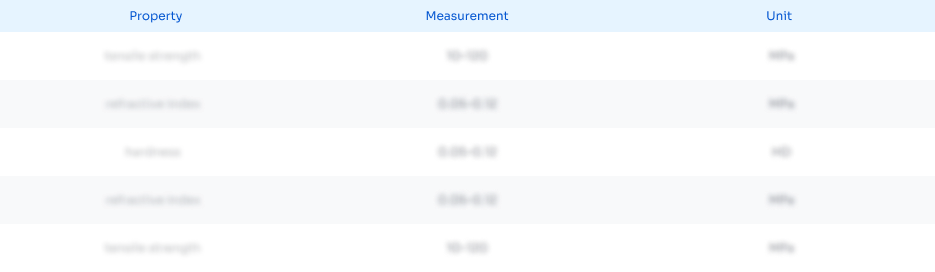
Abstract
Description
Claims
Application Information
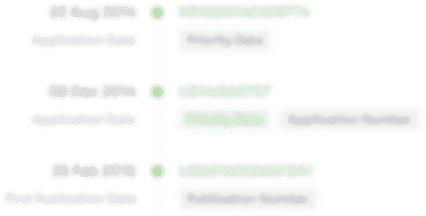
- R&D Engineer
- R&D Manager
- IP Professional
- Industry Leading Data Capabilities
- Powerful AI technology
- Patent DNA Extraction
Browse by: Latest US Patents, China's latest patents, Technical Efficacy Thesaurus, Application Domain, Technology Topic, Popular Technical Reports.
© 2024 PatSnap. All rights reserved.Legal|Privacy policy|Modern Slavery Act Transparency Statement|Sitemap|About US| Contact US: help@patsnap.com