An electric power equipment detection algorithm based on a convolution neural network
A technology of convolutional neural network and power equipment, applied in the direction of biological neural network model, neural learning method, neural architecture, etc., can solve the problems of difficult network convergence and low detection accuracy, and achieve excellent detection performance and real-time accurate detection effect
- Summary
- Abstract
- Description
- Claims
- Application Information
AI Technical Summary
Problems solved by technology
Method used
Image
Examples
Embodiment Construction
[0054] The present invention is further described below.
[0055] Implementation process and examples of the present invention are as follows:
[0056] (1) First, 5,000 images were acquired through the visible light camera and the thermal imaging camera, totaling 10,000 images. Taking visible light images as an example, divide 5000 visible light images into 4500 training sets and 500 test sets, and there is no intersection between the training set and the test set. The division of thermal imaging images is the same as that of visible light images. Then use the labelImg tool to label the image to obtain the labels about the power equipment in the image. The label of the electrical equipment contains the coordinates (x1, y1) of the upper left corner of the relevant electrical equipment in the image, the coordinates (x2, y2) of the lower right corner and the type c of the electrical equipment. In particular, there may be multiple different types of electrical equipment in an i...
PUM
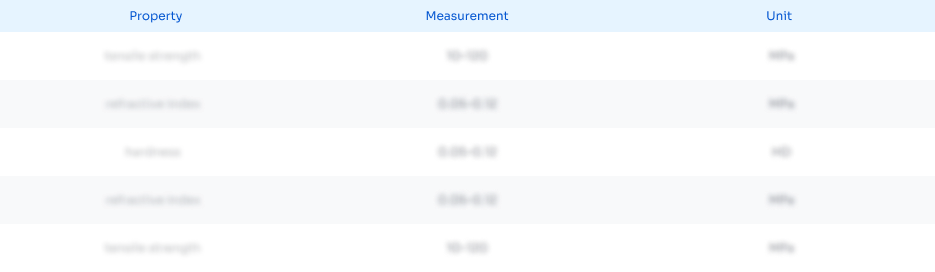
Abstract
Description
Claims
Application Information
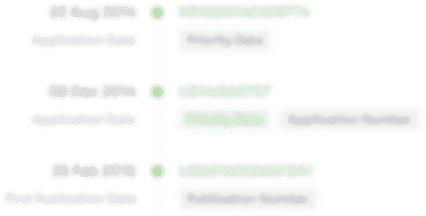
- R&D
- Intellectual Property
- Life Sciences
- Materials
- Tech Scout
- Unparalleled Data Quality
- Higher Quality Content
- 60% Fewer Hallucinations
Browse by: Latest US Patents, China's latest patents, Technical Efficacy Thesaurus, Application Domain, Technology Topic, Popular Technical Reports.
© 2025 PatSnap. All rights reserved.Legal|Privacy policy|Modern Slavery Act Transparency Statement|Sitemap|About US| Contact US: help@patsnap.com