A network representation learning method based on multi-order proximity similarity
A similarity and network technology, applied in the field of complex network analysis, to achieve the effect of rich semantics, high reliability and authenticity
- Summary
- Abstract
- Description
- Claims
- Application Information
AI Technical Summary
Problems solved by technology
Method used
Image
Examples
Embodiment Construction
[0041] Such as figure 1 As shown, the present invention discloses a network representation learning method based on multi-order proximity similarity, including:
[0042] (1) The real social network structure is abstracted into an undirected graph G(V,E), where V represents a user node, and E represents the follow-to-follow relationship between users.
[0043] (2) Take out a node A in the network, find out the adjacent nodes whose step size does not exceed k, and put these nodes into A's context node set S A middle. Each node has a corresponding set of context nodes in the following form: S A ={[B:NN AB ],[C:NN AC ],....,[Q:NN AQ ]},
[0044] Among them, [] represents the context node element, and the nodes in the element exist in the form of key-value pairs. The key of the element represents the name of the context node, and the value of the element is the degree of association between the context node and the original node.
[0045] (3) Initialize the context node set ...
PUM
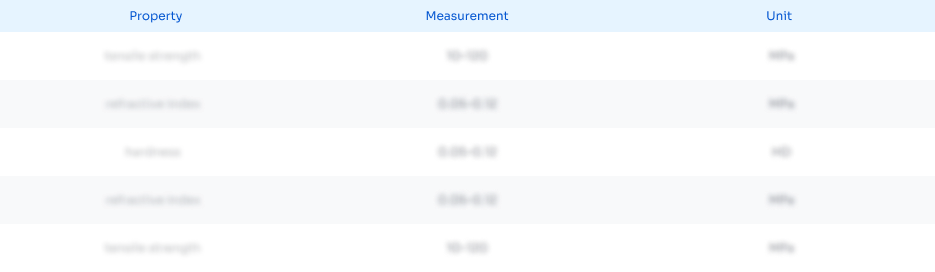
Abstract
Description
Claims
Application Information
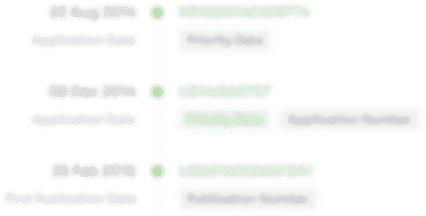
- R&D Engineer
- R&D Manager
- IP Professional
- Industry Leading Data Capabilities
- Powerful AI technology
- Patent DNA Extraction
Browse by: Latest US Patents, China's latest patents, Technical Efficacy Thesaurus, Application Domain, Technology Topic, Popular Technical Reports.
© 2024 PatSnap. All rights reserved.Legal|Privacy policy|Modern Slavery Act Transparency Statement|Sitemap|About US| Contact US: help@patsnap.com