An Unsupervised Object Recognition Approach Combining Multi-Source Feature Learning and Group Sparsity Constraints
A feature learning and object recognition technology, applied in the field of machine learning, can solve the problems of affecting the clustering effect, increasing the complexity of subspace search, consuming expensive computing resources, etc., achieving good anti-interference, saving computer resources, and improving accuracy The effect of sex and scalability
- Summary
- Abstract
- Description
- Claims
- Application Information
AI Technical Summary
Problems solved by technology
Method used
Image
Examples
Embodiment Construction
[0055] The technical solutions and beneficial effects of the present invention will be described in detail below in conjunction with the accompanying drawings.
[0056] Such as figure 1 and figure 2 As shown, the present invention provides an unsupervised object recognition method combining multi-source feature learning and group sparse constraints, including the following steps:
[0057] (1) Obtain V types of features (views) from the image set to be processed containing c categories, and form these features into a data set X=[x 1 ,x 2 ,...,x n ]∈R d×n , where d represents the feature dimension of the data, and n represents the number of samples in the data set.
[0058] (2) Extract the total scatter matrix S of the data set t :
[0059]
[0060] in, is the overall mean of the samples in the dataset.
[0061] (3) On the basis of step (2), construct a KM clustering model based on linear discriminant analysis, and the objective function is as follows:
[0062] ...
PUM
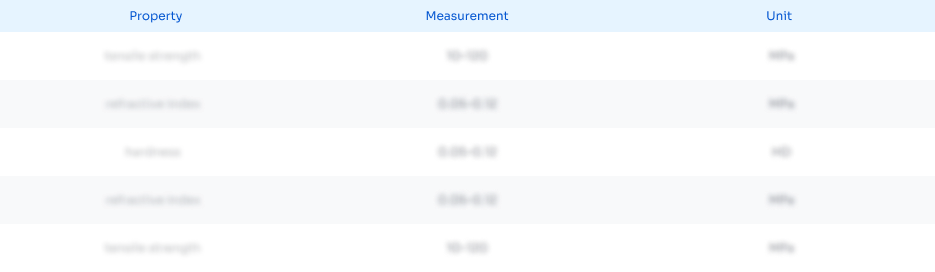
Abstract
Description
Claims
Application Information
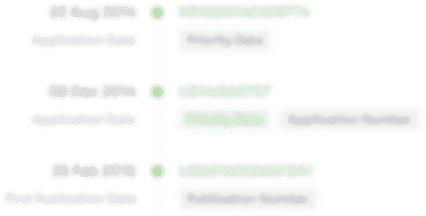
- R&D
- Intellectual Property
- Life Sciences
- Materials
- Tech Scout
- Unparalleled Data Quality
- Higher Quality Content
- 60% Fewer Hallucinations
Browse by: Latest US Patents, China's latest patents, Technical Efficacy Thesaurus, Application Domain, Technology Topic, Popular Technical Reports.
© 2025 PatSnap. All rights reserved.Legal|Privacy policy|Modern Slavery Act Transparency Statement|Sitemap|About US| Contact US: help@patsnap.com