An HARDI compressed sensing super-resolution reconstruction method based on deep dictionary learning
A technology of super-resolution reconstruction and dictionary learning, which is applied in image data processing, instrumentation, computing, etc., can solve problems such as weak dictionary expression ability, and achieve good nerve fiber reconstruction ability, less sampled data, and fast data sampling speed Effect
- Summary
- Abstract
- Description
- Claims
- Application Information
AI Technical Summary
Problems solved by technology
Method used
Image
Examples
Embodiment Construction
[0027] The following detailed description is given in conjunction with the accompanying drawings.
[0028] The original signal in the present invention refers to a high-resolution signal without down-sampling.
[0029] The signal to be reconstructed in the present invention refers to a low-resolution signal obtained after the sample is down-sampled by the measurement matrix.
[0030] figure 1 It is a schematic diagram of compressed sensing of a single-layer dictionary. like figure 1 As shown, x is the original signal. In practice, the acquisition time for acquiring the original signal is too long, which is inconvenient for acquisition. y is the signal to be reconstructed, that is, the actual measurement signal, α is the sparse signal, and Ψ and Φ are the dictionary and the measurement matrix, respectively.
[0031] The mathematical expression of single-layer compressed sensing is:
[0032] x=Ψ*α (1)
[0033] y=Φ*Ψ*α (2)
[0034] The data is compressed and sampled by mea...
PUM
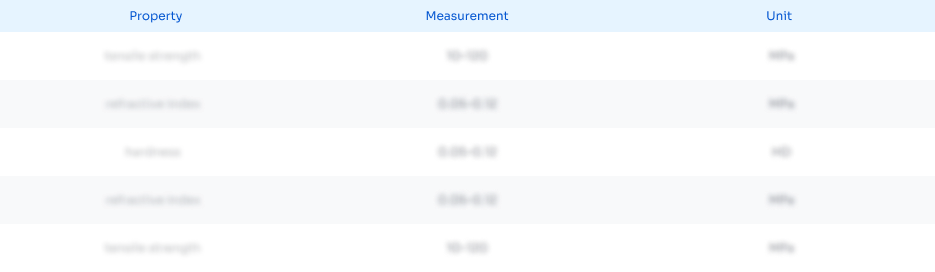
Abstract
Description
Claims
Application Information
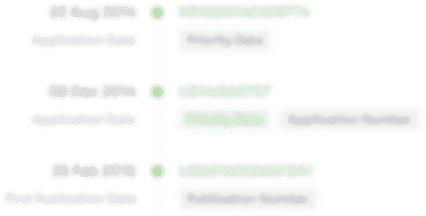
- R&D
- Intellectual Property
- Life Sciences
- Materials
- Tech Scout
- Unparalleled Data Quality
- Higher Quality Content
- 60% Fewer Hallucinations
Browse by: Latest US Patents, China's latest patents, Technical Efficacy Thesaurus, Application Domain, Technology Topic, Popular Technical Reports.
© 2025 PatSnap. All rights reserved.Legal|Privacy policy|Modern Slavery Act Transparency Statement|Sitemap|About US| Contact US: help@patsnap.com