A reinforcement learning tri-state combination long-short-term memory neural network system and a training and prediction method thereof
A long-short-term memory, neural network technology, applied in reinforcement learning three-state combined long-short-term memory neural network system and training and prediction fields, can solve the model prediction accuracy and calculation efficiency is not ideal, sample validity and diversity loss, prediction Uncertain results, etc., to avoid blind search, enhance generalization ability, and improve convergence speed
- Summary
- Abstract
- Description
- Claims
- Application Information
AI Technical Summary
Problems solved by technology
Method used
Image
Examples
Embodiment Construction
[0037] A three-state combined long-short-term memory neural network system for reinforcement learning, including a long-short-term memory neural network, a reinforcement learning unit and a monotone trend recognizer.
[0038] Long short-term memory neural network includes input gate, output gate, forget gate, memory unit and candidate memory unit, and unit output (ie hidden layer state).
[0039] The monotone trend recognizer judges the trend state of the time series constructed by the input, and the reinforcement learning unit selects a long-short-term memory neural network with the number of hidden layers and the number of nodes in the hidden layer that adapt to its changing law according to the trend state of the input time series. Among them, the trend state of the input time series includes three states, and each trend state corresponds to a long-short-term memory neural network whose number of hidden layers and hidden layer nodes are adapted to its changing law.
[0040]...
PUM
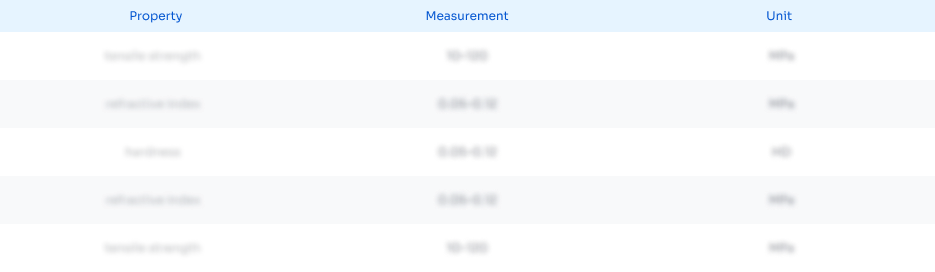
Abstract
Description
Claims
Application Information
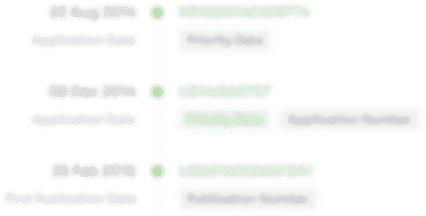
- R&D
- Intellectual Property
- Life Sciences
- Materials
- Tech Scout
- Unparalleled Data Quality
- Higher Quality Content
- 60% Fewer Hallucinations
Browse by: Latest US Patents, China's latest patents, Technical Efficacy Thesaurus, Application Domain, Technology Topic, Popular Technical Reports.
© 2025 PatSnap. All rights reserved.Legal|Privacy policy|Modern Slavery Act Transparency Statement|Sitemap|About US| Contact US: help@patsnap.com