Gait abnormality classification method based on deep convolution neural network
An abnormal gait and neural network technology, applied in medical science, sensors, diagnostic recording/measurement, etc., can solve problems such as high computational complexity and poor real-time performance, improve classification accuracy, save cycle division and feature extraction engineering , The effect of reducing the workload of data preprocessing
- Summary
- Abstract
- Description
- Claims
- Application Information
AI Technical Summary
Problems solved by technology
Method used
Image
Examples
Embodiment Construction
[0026] The present invention will be described in detail below with reference to the accompanying drawings and preferred embodiments.
[0027] The invention provides a method for classifying human motion gait based on a convolutional neural network, which is implemented by the following steps:
[0028] Step 1: Fix the IMU hardware system on the outer side of the right calf through a strap, where the Y-axis is perpendicular to the horizontal plane, the X-axis is perpendicular to the coronal plane of the human body, and the Z-axis is perpendicular to the sagittal plane of the human body. Set the system sampling rate to 512Hz, set the IMU accelerometer lead rate to ±2g, collect the motion signal of the human body during normal walking, and collect no less than 100 steps to obtain the normal gait three-axis acceleration information. An example of the original signal (intercepted 10s) such as figure 2 shown.
[0029] Step 2, the IMU placement and collection process is the same a...
PUM
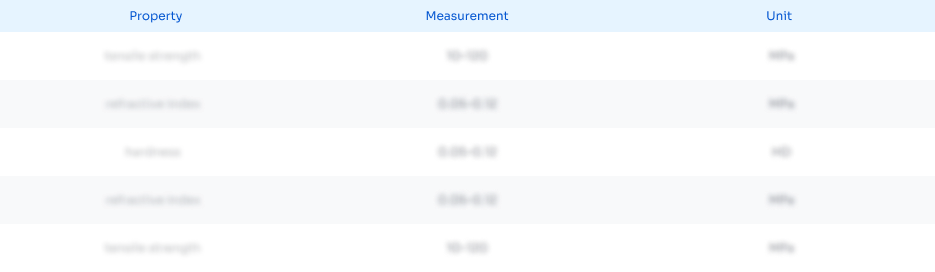
Abstract
Description
Claims
Application Information
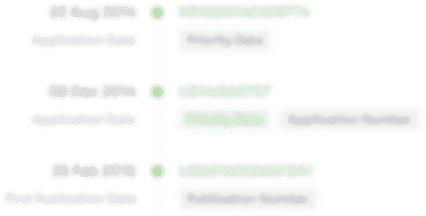
- R&D Engineer
- R&D Manager
- IP Professional
- Industry Leading Data Capabilities
- Powerful AI technology
- Patent DNA Extraction
Browse by: Latest US Patents, China's latest patents, Technical Efficacy Thesaurus, Application Domain, Technology Topic, Popular Technical Reports.
© 2024 PatSnap. All rights reserved.Legal|Privacy policy|Modern Slavery Act Transparency Statement|Sitemap|About US| Contact US: help@patsnap.com