A power system dynamic safety confidence evaluation method based on an integrated learning scheme
A power system and integrated learning technology, applied in integrated learning, instruments, data processing applications, etc., can solve problems such as weak robustness and generalization ability, weakening DSA strength, classification or prediction errors and inaccurate DSA results, etc. Achieve the effect of enhancing the robustness and generalization ability of the system, improving the stability of the system structure, and benefiting the stability of the model structure
- Summary
- Abstract
- Description
- Claims
- Application Information
AI Technical Summary
Problems solved by technology
Method used
Image
Examples
Embodiment 1
[0111] Table 1 describes the system calculation time and performance table obtained by testing the present invention on the IEEE-50 computer system. The system consists of 50 generators, 145 nodes and 453 branches. In the following test, 200 ELMs are used and corresponding parameters are set, 3000 samples and 30 features are randomly selected, the credibility estimation parameter is set to 40, 20% of the database is randomly selected as the test data, and the rest as training data. Consider a single contingency case first, and test multiple contingency cases last. The average training and testing time of a single ELM during validation is only 1.54 seconds and 0.0313 seconds, respectively. IS is fully tested and shown, as shown in Table 1: the confidence is 93.85%, and the accuracy is 100%, that is, 1191 out of 1269 instances can be reliably determined by IS, and their classification is 100% correct, which means IS can detect potential misclassification very well.
[0112] ...
experiment example 2
[0116] Table 2 tests the calculation time and performance of the dynamic equivalent system of China's real-world power grid. There are 39 motors, 120 load nodes, 223 AC lines and 4 high-voltage DC transmission lines. In this test, 784 operation samples and 196 candidate features are selected, 20% of the database is randomly selected as test data, and the rest are used as training data. There are 157 test instances and 627 training instances respectively, and the training data are used to adjust Single ELM. Finally, the comprehensive test results are shown in Table 2, which also confirms the Figure 5 As shown by the regularity, IS can well identify prediction errors.
[0117] Table 2
[0118]
PUM
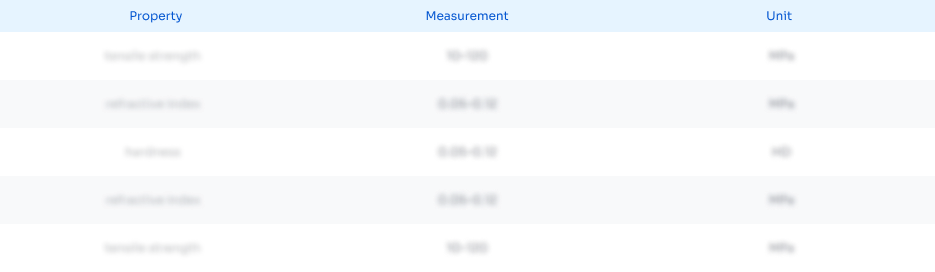
Abstract
Description
Claims
Application Information
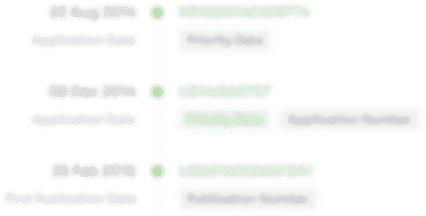
- R&D Engineer
- R&D Manager
- IP Professional
- Industry Leading Data Capabilities
- Powerful AI technology
- Patent DNA Extraction
Browse by: Latest US Patents, China's latest patents, Technical Efficacy Thesaurus, Application Domain, Technology Topic, Popular Technical Reports.
© 2024 PatSnap. All rights reserved.Legal|Privacy policy|Modern Slavery Act Transparency Statement|Sitemap|About US| Contact US: help@patsnap.com