A fault identification method of rolling bearing under variable working conditions based on att-cnn
A technology of ATT-CNN and rolling bearings, applied in mechanical bearing testing, geometric CAD, biological neural network models, etc., can solve problems such as generalization ability constraints
- Summary
- Abstract
- Description
- Claims
- Application Information
AI Technical Summary
Problems solved by technology
Method used
Image
Examples
experiment approach 2
[0140] In order to verify the generalization ability of the algorithm proposed in this paper, experiments are carried out on data of all load types. All state data sets under two loads are used as the training set, and the data under the other two different loads are used as the test set. The data settings and experimental results are shown in Table 4. The structure of multi-condition type data is more complex and diverse, and it is more difficult to identify the fault state when testing and diagnosing single-condition data.
[0141] Table 4 Experimental scheme 2 data set settings and accuracy comparison
[0142]
[0143] Through the analysis of multiple sets of experiments, the average test accuracy of the ATT-CNN model is 96.48%, which is 3.3% higher than that of CNN. It shows that under more complex working conditions, the data structure and distribution of the test set and the training set are quite different. The ATT-CNN model can well adapt to changes in data distrib...
experiment approach 3
[0146] In order to further verify the robustness of the model, the data under one load is used as the training set, and the data under the other three loads is used as the test set. The data settings and experimental results are shown in Table 5. As we all know, for deep neural networks, a more robust model can be obtained by using massive training data. However, in practical applications, some working condition data are difficult to obtain or unknown working condition data may appear. Therefore, it is hoped that a small data set can be used to realize fault diagnosis under variable working conditions.
[0147] Table 5 Experimental scheme three data set settings and accuracy comparison
[0148]
[0149]
[0150] The average test accuracy of the ATT-CNN-based model is 83.40%, while the unimproved CNN model is only 77.98%, which is 5.5% higher. The overall performance of the proposed algorithm on the dataset set by scheme three is not as good as that of the first two sche...
PUM
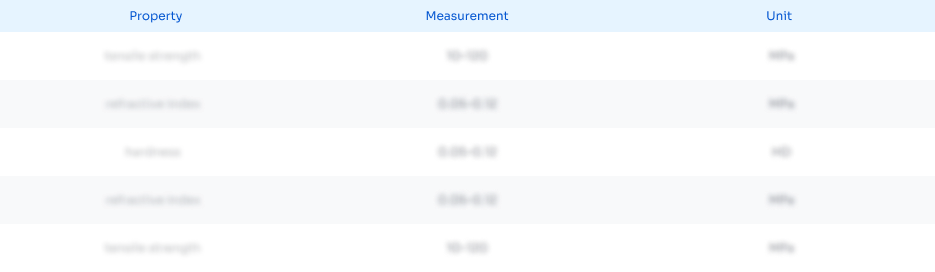
Abstract
Description
Claims
Application Information
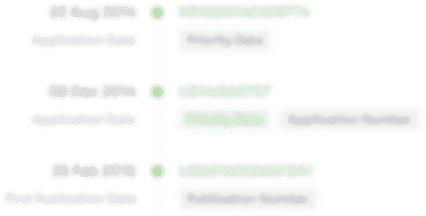
- Generate Ideas
- Intellectual Property
- Life Sciences
- Materials
- Tech Scout
- Unparalleled Data Quality
- Higher Quality Content
- 60% Fewer Hallucinations
Browse by: Latest US Patents, China's latest patents, Technical Efficacy Thesaurus, Application Domain, Technology Topic, Popular Technical Reports.
© 2025 PatSnap. All rights reserved.Legal|Privacy policy|Modern Slavery Act Transparency Statement|Sitemap|About US| Contact US: help@patsnap.com