Hyperspectral unknown category target detection method based on a probability model and deep learning
A probabilistic model and deep learning technology, applied in the field of hyperspectral target detection of unknown categories, can solve the problems of low detection and recognition accuracy, long running time, and complex calculations
- Summary
- Abstract
- Description
- Claims
- Application Information
AI Technical Summary
Problems solved by technology
Method used
Image
Examples
Embodiment Construction
[0044] The present invention will be further described in detail below in conjunction with the accompanying drawings and specific implementation examples.
[0045] figure 1 It shows the overall flow chart of object detection of unknown category. The hyperspectral training data is input to the trained CNN classification model, and the output of the last fully connected layer of the CNN classification model is used as the activation vector of each category of samples. The activation vectors of the correctly classified samples in each category are accumulated and the mean is calculated, and the category is represented by the mean activation vector. Use the activation vector and the mean activation vector of each category to fit the Weibull probability model belonging to each category, and the fitting result is the position parameter τ of the Weibull probability model j , scale parameter λ j , shape parameters etc. κ j . Based on the Weibull fitting results of each category, t...
PUM
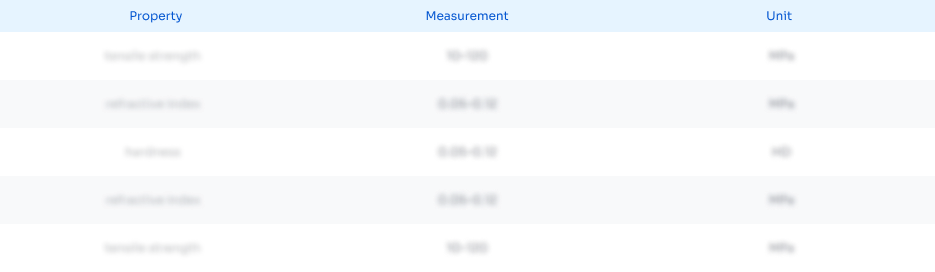
Abstract
Description
Claims
Application Information
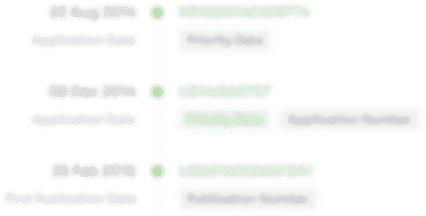
- R&D Engineer
- R&D Manager
- IP Professional
- Industry Leading Data Capabilities
- Powerful AI technology
- Patent DNA Extraction
Browse by: Latest US Patents, China's latest patents, Technical Efficacy Thesaurus, Application Domain, Technology Topic.
© 2024 PatSnap. All rights reserved.Legal|Privacy policy|Modern Slavery Act Transparency Statement|Sitemap