An image super-resolution reconstruction method based on a dense feature fusion network
A technology of super-resolution reconstruction and feature fusion, applied in image enhancement, image data processing, graphics and image conversion, etc., can solve the problems of loss of low-resolution image details, increased computing load, artifacts, etc., and achieve high resolution Restore image details, improve quality and accuracy, and reduce noise
- Summary
- Abstract
- Description
- Claims
- Application Information
AI Technical Summary
Problems solved by technology
Method used
Image
Examples
Embodiment Construction
[0027] The present invention will be further described below in conjunction with the embodiments and accompanying drawings, but the present invention is not limited thereto.
[0028] Examples of the present invention figure 1 The following steps are shown:
[0029] 1) Data preprocessing: the original color image is numerically normalized to [0,1], and the original color image is interpolated and scaled according to different magnification ratios to generate data sets with different magnification ratios for subsequent model training .
[0030] 2) Establish reconstruction model: such as figure 2 As shown, the reconstruction model includes a coarse feature extraction network, a dense feature fusion network and an image reconstruction network. The coarse feature extraction network consists of a layer of convolutional neural network for extracting coarse features F directly from the original low-resolution image 0 , whose number of features is 32.
[0031] f 0 =W coarse ×Im...
PUM
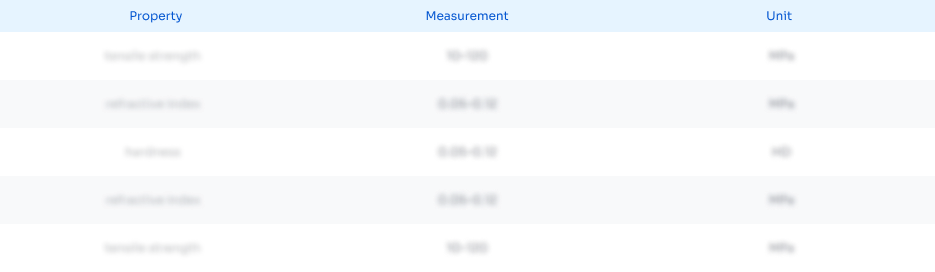
Abstract
Description
Claims
Application Information
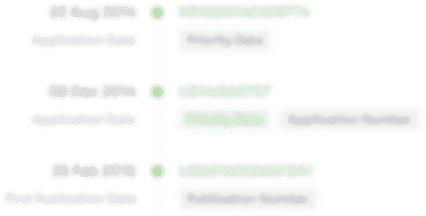
- Generate Ideas
- Intellectual Property
- Life Sciences
- Materials
- Tech Scout
- Unparalleled Data Quality
- Higher Quality Content
- 60% Fewer Hallucinations
Browse by: Latest US Patents, China's latest patents, Technical Efficacy Thesaurus, Application Domain, Technology Topic, Popular Technical Reports.
© 2025 PatSnap. All rights reserved.Legal|Privacy policy|Modern Slavery Act Transparency Statement|Sitemap|About US| Contact US: help@patsnap.com