Real-time equipment abnormality detection device and method based on time sequence prediction model
A technology for time series prediction and equipment abnormality. It is applied in prediction, calculation model, instrument, etc. It can solve the problems of deviation or error of abnormal state judgment results, excessive dependence on operation, inability to apply, etc., and achieve the effect of high model accuracy.
- Summary
- Abstract
- Description
- Claims
- Application Information
AI Technical Summary
Problems solved by technology
Method used
Image
Examples
Embodiment 1
[0019] The real-time device anomaly detection device based on the timing prediction model in this embodiment specifically includes: a data layer, a logic control layer, a model center, and a display layer; the data layer includes a real-time database, a data buffer, a historical database, and a timing data processing module. The logic control layer includes a trainer and a predictor; the model center includes a machine learning model; the display layer includes a result display module.
[0020] In this embodiment, the real-time database is used to store data generated during the operation of each device in the production system; wherein, the data generated during the operation of each device in the production system is collected by a data collection device. In this embodiment, the data buffer is used to cache the data of the preset time window; the process of running the time series prediction model needs to transfer the data of a certain time window to the predictor, so a data...
Embodiment 2
[0024] Such as image 3 as shown, image 3 It is a schematic diagram of the real-time device anomaly detection framework based on the time series prediction model of the present invention. The labels ①-⑧ in the figure correspond to S1011, S1012, S1021, S1022, S1023, S1024, S1031, and S1032 below, respectively.
[0025] This embodiment provides a real-time equipment anomaly detection method based on a time series prediction model. The method is implemented based on the above-mentioned real-time equipment anomaly detection device based on a time series prediction model, and includes the following steps:
[0026] S101. Initial model training: S1011. Import the historical data of each device in the production system into the time series data processing module for processing; S1012. The time series prediction model is trained according to the time series data processed by the time series data processing module, and the trained time series Predictive models are saved to the Model C...
PUM
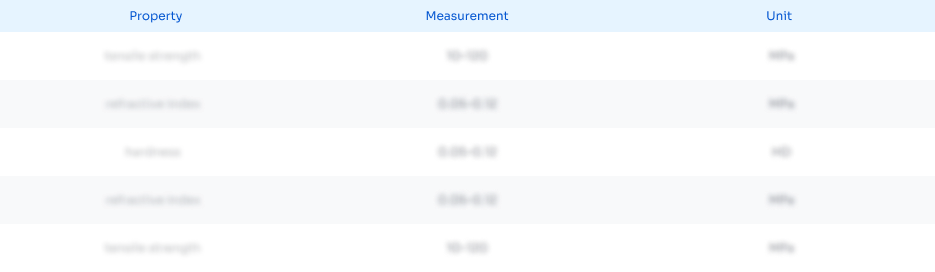
Abstract
Description
Claims
Application Information
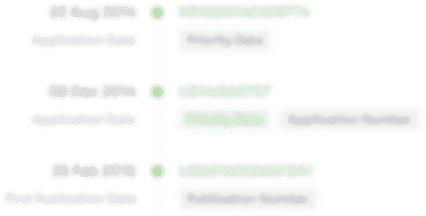
- R&D
- Intellectual Property
- Life Sciences
- Materials
- Tech Scout
- Unparalleled Data Quality
- Higher Quality Content
- 60% Fewer Hallucinations
Browse by: Latest US Patents, China's latest patents, Technical Efficacy Thesaurus, Application Domain, Technology Topic, Popular Technical Reports.
© 2025 PatSnap. All rights reserved.Legal|Privacy policy|Modern Slavery Act Transparency Statement|Sitemap|About US| Contact US: help@patsnap.com