Image super-resolution reconstruction method based on convolutional neural network
A convolutional neural network and super-resolution reconstruction technology, applied in the field of image super-resolution reconstruction based on convolutional neural network, can solve the problem that the three-layer structure cannot meet the fineness and other problems
- Summary
- Abstract
- Description
- Claims
- Application Information
AI Technical Summary
Problems solved by technology
Method used
Image
Examples
Embodiment Construction
[0030] The present invention will be further described in detail below in conjunction with the accompanying drawings and embodiments.
[0031] The present invention includes the following processes:
[0032] First convert the image of the RGB channel to the image of the YCbCr channel, and reconstruct each channel; then design an eight-layer convolutional neural network structure, determine the size and number of convolution kernels in each layer, and optimize the CNN, including Optimization of the initialization parameters weights and bias, and optimization of the activation function; finally, the shallow feature information is learned through the four-layer network of SRCNN, and migrated to the TLSRCNN proposed by the present invention, and the final four-layer network strengthens the salient features to complete the final image super Resolution reconstruction.
[0033] Such as figure 1 Shown is the eight-layer end-to-end neural network model structure diagram based on feat...
PUM
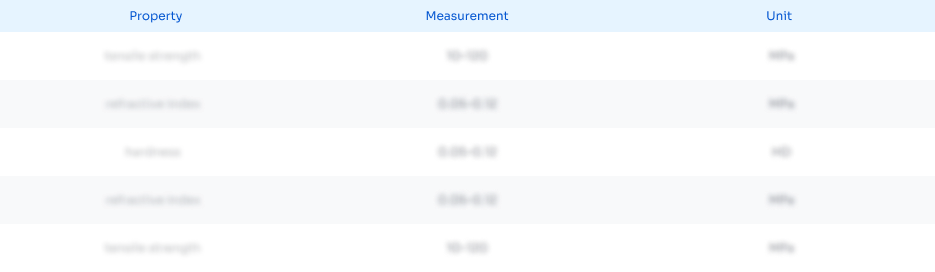
Abstract
Description
Claims
Application Information
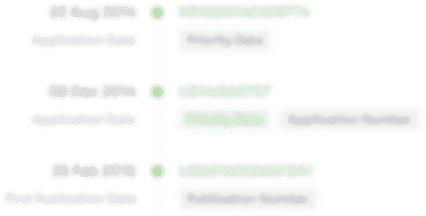
- R&D Engineer
- R&D Manager
- IP Professional
- Industry Leading Data Capabilities
- Powerful AI technology
- Patent DNA Extraction
Browse by: Latest US Patents, China's latest patents, Technical Efficacy Thesaurus, Application Domain, Technology Topic, Popular Technical Reports.
© 2024 PatSnap. All rights reserved.Legal|Privacy policy|Modern Slavery Act Transparency Statement|Sitemap|About US| Contact US: help@patsnap.com