Super-resolution reconstruction method based on conditional generative adversarial network
A technology of super-resolution reconstruction and conditional generation, which is applied in the field of image processing to achieve the effect of improving the discrimination accuracy, improving the accuracy of the model, and shortening the running time.
- Summary
- Abstract
- Description
- Claims
- Application Information
AI Technical Summary
Problems solved by technology
Method used
Image
Examples
Embodiment Construction
[0040] Embodiments of the invention are described in detail below, examples of which are illustrated in the accompanying drawings. The embodiments described below by referring to the figures are exemplary only for explaining the present invention and should not be construed as limiting the present invention.
[0041] Such as figure 1 As shown, the flow chart of the super-resolution reconstruction method based on the conditional generation confrontation network provided by the present invention, the specific steps are as follows:
[0042]Step 1: Downsample the high-resolution training image to obtain a low-resolution training image. Specifically: the training set uses the VOC2012 data set, and of course the present invention is also applicable to other training sets. The algorithm used for downsampling from high resolution to low resolution is the bicubic interpolation algorithm. Second, corresponding random cropping of high and low resolution images is required. The crop s...
PUM
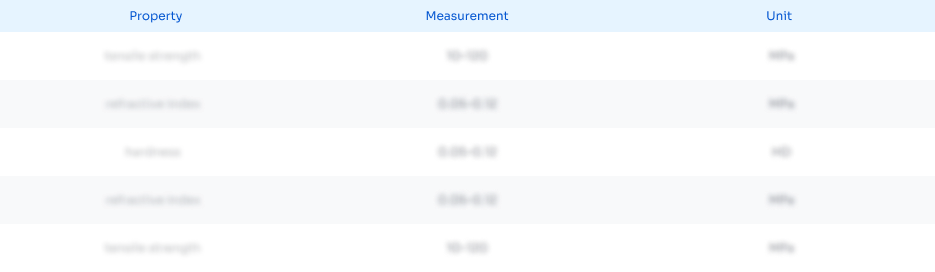
Abstract
Description
Claims
Application Information
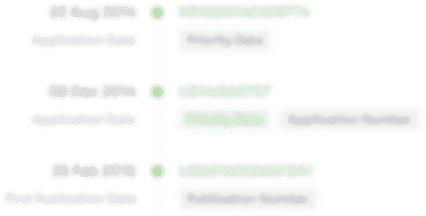
- R&D Engineer
- R&D Manager
- IP Professional
- Industry Leading Data Capabilities
- Powerful AI technology
- Patent DNA Extraction
Browse by: Latest US Patents, China's latest patents, Technical Efficacy Thesaurus, Application Domain, Technology Topic.
© 2024 PatSnap. All rights reserved.Legal|Privacy policy|Modern Slavery Act Transparency Statement|Sitemap