Improved self-adaptive sparse sampling fault classification method
A sparse sampling and fault classification technology, applied in the fields of instrumentation, calculation, mechanical bearing testing, etc., can solve problems such as rotating machinery fault classification, and achieve the effect of solving time-shift deviation, reducing redundant information, and improving computing efficiency.
- Summary
- Abstract
- Description
- Claims
- Application Information
AI Technical Summary
Problems solved by technology
Method used
Image
Examples
Embodiment Construction
[0046] (1) Acquisition of vibration signals: The vibration signals of bearings are collected by means of the rotating machinery failure simulation experiment platform. The bearing defects, sampling frequency, spindle speed, and signal length can be set by yourself. In the case of the same sampling frequency and spindle speed, the vibration signals of normal bearing, inner ring fault, outer ring fault, and rolling element fault are collected respectively, and four types of signal test samples and training samples are respectively constructed, and the length of each group of signals is N. Normalize the signal to ensure the unity of signal amplitude and magnitude;
[0047] (2) Feature enhancement: use wavelet modulus maxima to perform sparse representation feature enhancement processing on training sample signals and test sample signals, and extract fault features. The training sample signal and the test sample signal are decomposed by t-layer wavelet respectively to obtain the w...
PUM
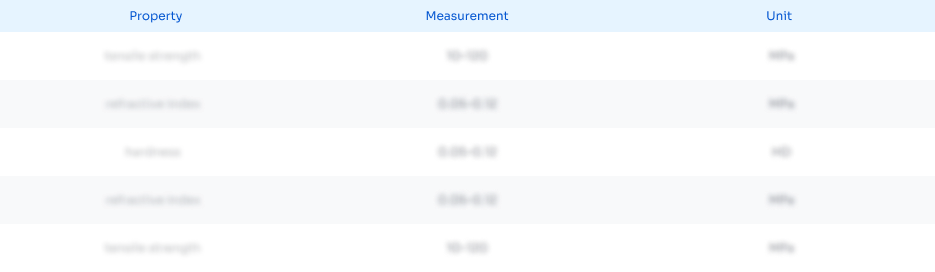
Abstract
Description
Claims
Application Information
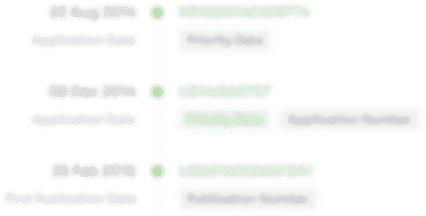
- R&D Engineer
- R&D Manager
- IP Professional
- Industry Leading Data Capabilities
- Powerful AI technology
- Patent DNA Extraction
Browse by: Latest US Patents, China's latest patents, Technical Efficacy Thesaurus, Application Domain, Technology Topic.
© 2024 PatSnap. All rights reserved.Legal|Privacy policy|Modern Slavery Act Transparency Statement|Sitemap