Automatic arrhythmia classifying method based on discriminant deep belief network
A deep belief network, arrhythmia technology, applied in the field of arrhythmia detection and classification, can solve problems such as validity impact
- Summary
- Abstract
- Description
- Claims
- Application Information
AI Technical Summary
Problems solved by technology
Method used
Image
Examples
specific Embodiment approach 1
[0080] A method for automatically classifying cardiac arrhythmias based on a discriminative deep belief network, the method comprising the steps of:
[0081] Step 1: ECG signal preprocessing;
[0082] Before the ECG signal is sent to the DDBNs network, it needs to be filtered, R peak location and normalization;
[0083] Step 2: DDBNs model construction;
[0084] (1) GRBM
[0085] DBNs is a graphical model that learns to extract deep representations of training data. It consists of stacked RBMs. RBMs are typical neural networks with visible and hidden layer interconnections. There is no connection between any two neurons in the same layer. An RBM with a binary input on the visible layer is a BB-RBM, while an RBM with a real-valued input on the visible layer is a GB-RBM;
[0086] (2) DRBM;
[0087] DRBM uses a single RBM with two sets of visible layers to train the joint density model. In addition to the unit A representing the input data, there is also a classification labe...
specific Embodiment approach 2
[0096] This embodiment is a further description of the discriminative deep belief network-based automatic arrhythmia classification method described in the first embodiment, and the first step includes the following process:
[0097] Firstly, the signal is decomposed by 9-scale wavelet, the detail coefficient of the first layer is 90-180 Hz and the approximation coefficient of the ninth layer is 0-0.35 Hz, and the other wavelet coefficients are reconstructed after adaptive wavelet threshold filtering to remove baseline drift and High frequency interference;
[0098] Then, determine the heart beat position by R peak positioning;
[0099] Finally, 256 sampling points were selected for the heart beat length including P wave and T wave, that is, 90 sampling points before and 165 sampling points after R peak were taken.
specific Embodiment approach 3
[0101] This embodiment is a further description of the arrhythmia automatic classification method based on the discriminative deep belief network described in the first embodiment, and the specific process of the step 2 GRBM is as follows:
[0102] The concrete process of described step two GRBM is:
[0103] DBNs is a graphical model that learns to extract deep representations of training data. It consists of stacked RBMs. RBMs are typical neural networks with visible and hidden layer interconnections. There is no connection between any two neurons in the same layer. The RBM with binary input on the visible layer is BB-RBM, and the RBM with real-valued input on the visible layer is GB-RBM. The energy functions of BB-RBM and GB-RBM are defined as formula (1) and formula ( 2) as shown:
[0104]
[0105]
[0106] Among them, θ 1 ={w ij ,b i ,c j}, θ 2 ={w ij ,b i ,c j ,σ j} represents the RBM parameters to be trained;
[0107] no v and n h are the number of visib...
PUM
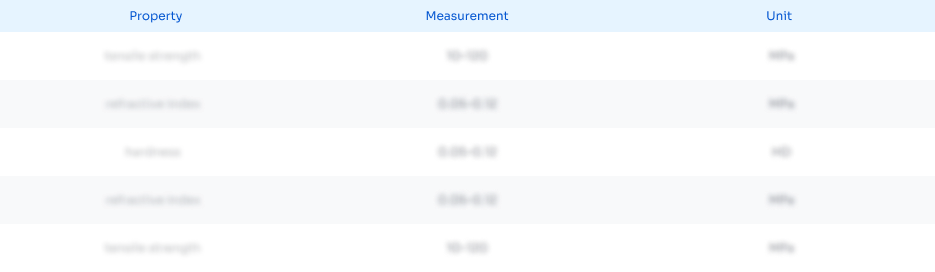
Abstract
Description
Claims
Application Information
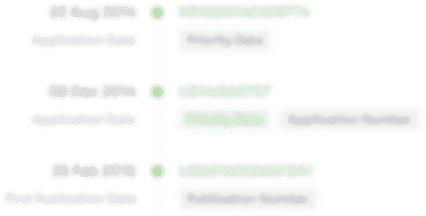
- R&D Engineer
- R&D Manager
- IP Professional
- Industry Leading Data Capabilities
- Powerful AI technology
- Patent DNA Extraction
Browse by: Latest US Patents, China's latest patents, Technical Efficacy Thesaurus, Application Domain, Technology Topic.
© 2024 PatSnap. All rights reserved.Legal|Privacy policy|Modern Slavery Act Transparency Statement|Sitemap