Supervised image classification method based on self-paced constraint mechanism
A classification method and image technology, applied in computer parts, instruments, character and pattern recognition, etc., can solve the problems of neglect and improve the effect of image recognition
- Summary
- Abstract
- Description
- Claims
- Application Information
AI Technical Summary
Problems solved by technology
Method used
Image
Examples
Embodiment 1
[0048] refer to figure 1 , provides a schematic diagram of the overall structure of a supervised image classification method based on a self-paced constraint mechanism, such as figure 1 , a supervised image classification method based on a self-procedural constraint mechanism, including S1: dividing training samples into difficult and easy categories; S2: establishing a sparse representation model, and bringing samples into the sparse representation model for training; S3: obtaining an image classification model and a prediction model; And, S4: constructing a category decision maker; wherein, the types of difficulty and difficulty of training samples include training easy samples and training difficult samples.
[0049] Specifically, the main structure of the present invention includes S1: Divide the difficulty types of training samples, the operator divides the database images, and divides them into training samples and test samples, and here divides the difficulty of the tra...
Embodiment 2
[0057] refer to figure 2 , this embodiment is different from the first embodiment in that: the step of obtaining the image classification model and the prediction model includes: S41: training and training easy samples; S42: updating X; S43: obtaining sparse code X, coefficient code D and self-stepping Constrained weighting coefficient α; S44: Determine the image classification model and prediction model. Specifically, see figure 1 , its main structure includes S1: Divide the difficulty types of training samples, the operator divides the database images into training samples and test samples, and here divides the difficulty of training samples, among which, the difficulty of training samples is divided into Training easy samples and training hard samples; S2: Establish a sparse representation model. It should be noted that the sparse representation model is a model related to self-paced constraint regularization, and the discriminant training easy samples and training diffic...
Embodiment 3
[0110] refer to Figure 4 , This embodiment is different from the above embodiments in that this embodiment relates to the technical field of plant image classification and recognition, specifically a supervised plant image classification method based on a self-paced constraint mechanism. Specifically, see figure 1 , its main structure includes S1: Divide the difficulty types of training samples, the operator divides the database images into training samples and test samples, and here divides the difficulty of training samples, among which, the difficulty of training samples is divided into Training easy samples and training hard samples; S2: Establish a sparse representation model. It should be noted that the sparse representation model is a model related to self-paced constraint regularization, and the discriminant training easy samples and training difficult samples are brought into the sparse representation in turn. Training in the model, it needs to be emphasized that th...
PUM
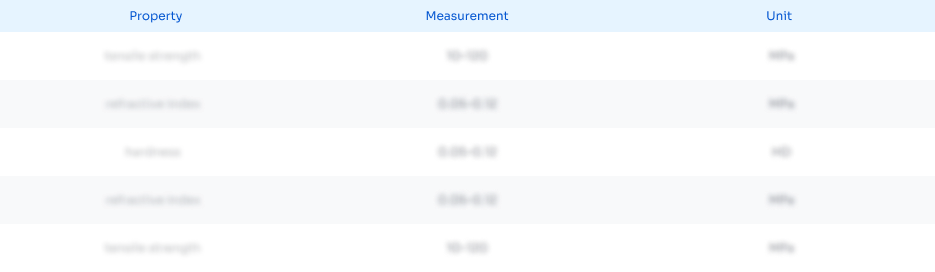
Abstract
Description
Claims
Application Information
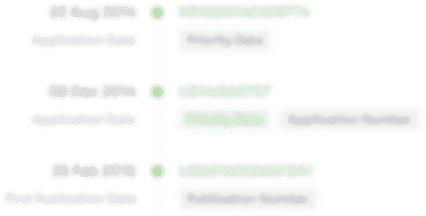
- R&D Engineer
- R&D Manager
- IP Professional
- Industry Leading Data Capabilities
- Powerful AI technology
- Patent DNA Extraction
Browse by: Latest US Patents, China's latest patents, Technical Efficacy Thesaurus, Application Domain, Technology Topic, Popular Technical Reports.
© 2024 PatSnap. All rights reserved.Legal|Privacy policy|Modern Slavery Act Transparency Statement|Sitemap|About US| Contact US: help@patsnap.com