Feature-weight-based LARS diabetes prediction method
A prediction method and technology for diabetes, applied in the field of medical informatization, can solve problems such as low accuracy, difficulty in finding key features directly for support vector machine prediction models, difficulty in traditional prediction methods for diabetes, etc., to achieve the effect of improving accuracy
- Summary
- Abstract
- Description
- Claims
- Application Information
AI Technical Summary
Problems solved by technology
Method used
Image
Examples
Embodiment Construction
[0049] Below in conjunction with the accompanying drawings and examples, the specific implementation of the present invention will be further described in detail, the following examples are used to illustrate the present invention, but not to limit the scope of the present invention.
[0050] It can be seen from machine learning and PCA theory that there are usually a few key features or principal components in a multidimensional sample; there are also only a few key features among the many features of diabetes. The study found that the LARS algorithm can be used to obtain the key features. A predictive model with better generalization ability; in addition, combining the feature weights obtained by the PCA algorithm in the solution step of the LARS algorithm can speed up the algorithm's approach to key features, thereby speeding up the algorithm's solution speed and accuracy.
[0051] First define the feature matrix of the diabetes dataset:
[0052]
[0053] That is, a matr...
PUM
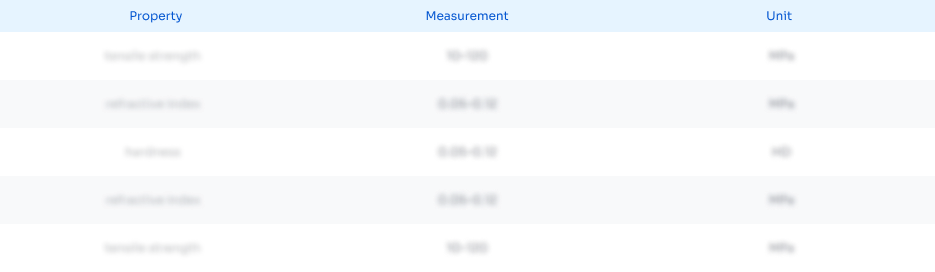
Abstract
Description
Claims
Application Information
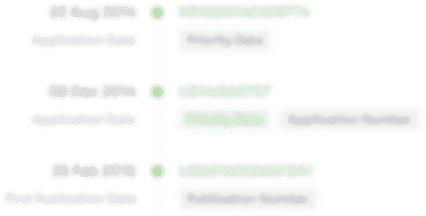
- Generate Ideas
- Intellectual Property
- Life Sciences
- Materials
- Tech Scout
- Unparalleled Data Quality
- Higher Quality Content
- 60% Fewer Hallucinations
Browse by: Latest US Patents, China's latest patents, Technical Efficacy Thesaurus, Application Domain, Technology Topic, Popular Technical Reports.
© 2025 PatSnap. All rights reserved.Legal|Privacy policy|Modern Slavery Act Transparency Statement|Sitemap|About US| Contact US: help@patsnap.com