Brain function network classification method based on deep forest
A technology of brain function network and classification method, applied in the field of non-neural network deep learning theory and application research, can solve the problems of restricted development and wider application, difficulty in hyperparameter adjustment, easy overfitting, etc., and achieve short training time. , the model generalization is good, the effect of alleviating the overfitting problem
- Summary
- Abstract
- Description
- Claims
- Application Information
AI Technical Summary
Problems solved by technology
Method used
Image
Examples
Embodiment Construction
[0018] We selected 30 brain regions located in the cerebral cortex from the AAL brain atlas as ROIs, and measured the statistical relationship between the neural activity signals of the brain regions by calculation methods such as Pearson correlation, partial correlation, and synchronization likelihood, and obtained The 30×30 brain function network adjacency matrix is used as an example as the input of the brain function network classification method based on deep forest. The basic structure of the method is as follows figure 1 As shown, its specific implementation steps are as follows:
[0019] Step (1): Initialization parameters: including parameters related to multi-granularity scanning and parameters related to cascading forest structure. The parameters of multi-granularity scanning include the granularity number k=3, the window size is m 1 =10,m 2 =15,m 3 =20, the type MC of the forest is random forest, the number of forests MN=2, the number of trees contained in eac...
PUM
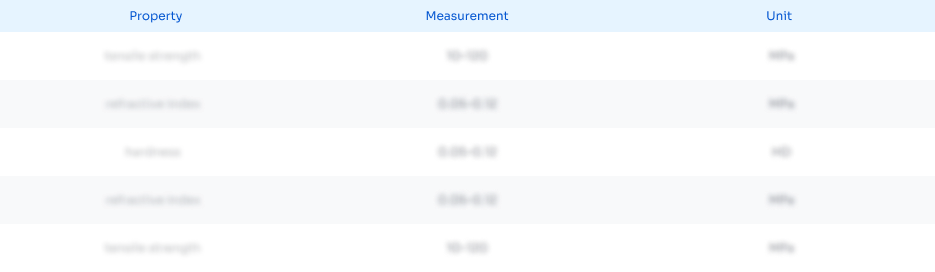
Abstract
Description
Claims
Application Information
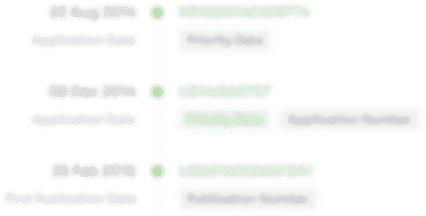
- R&D
- Intellectual Property
- Life Sciences
- Materials
- Tech Scout
- Unparalleled Data Quality
- Higher Quality Content
- 60% Fewer Hallucinations
Browse by: Latest US Patents, China's latest patents, Technical Efficacy Thesaurus, Application Domain, Technology Topic, Popular Technical Reports.
© 2025 PatSnap. All rights reserved.Legal|Privacy policy|Modern Slavery Act Transparency Statement|Sitemap|About US| Contact US: help@patsnap.com