An Action Recognition Method Based on Inductive Deep Learning
A technology of action recognition and deep learning, applied in character and pattern recognition, instruments, computing, etc., can solve the problems of large amount of training data and poor performance, achieve good recognition ability, improve recognition rate, and improve the effect of recognition
- Summary
- Abstract
- Description
- Claims
- Application Information
AI Technical Summary
Problems solved by technology
Method used
Image
Examples
Embodiment 1
[0089] An action recognition method based on inductive deep learning (such as figure 1 shown), including the following steps
[0090] Step 1: Use the camera to obtain a video sequence for action recognition;
[0091] Step 2: Preprocessing the video sequence to obtain one or more information source sequences with different characteristics;
[0092] Step 3: Select one or more types of features and construct a deep learning network that extracts the selected types of features;
[0093] Step 4: Input the information source sequence into the deep learning network to extract features, and obtain the action type of the video sequence.
[0094] The present invention is described from six aspects below:
[0095] 1. Selection of information sources. Based on action recognition, in the acquisition of information sources, grayscale images, RGB images and depth images are selected as three types of basic information sources. At the same time, extract the binarized image and histogram ...
Embodiment 2
[0102] This embodiment describes the present invention in detail based on Embodiment 1.
[0103] Step 1: Use the camera to obtain a video sequence for action recognition. The video sequence is an RGB action sequence. The recording scene of the camera is a variety of scenes. Actions may occur indoors or outdoors. In each scene, the camera Record the whole person.
[0104] Step 2: Preprocessing the video sequence to obtain one or more information source sequences with different characteristics;
[0105] In the process of action recognition, the video sequence is the RGB action sequence acquired by the camera without depth information, so the information source is the down-sampled RGB video stream and the position-corrected human joint point information stream;
[0106] Among them, the purpose of downsampling the video sequence is to reduce the calculation amount of the subsequent network, which is an existing technology; the human body joint point information flow is composed o...
Embodiment 3
[0134] This embodiment is used to illustrate the process of training the deep learning network architecture and using the trained deep learning network architecture to identify in the present invention.
[0135] Training process:
[0136] The first step: Divide the data set used for training into three parts, namely training set, verification set and test set;
[0137] Step 2: Use the training set to train the recognition structure separately;
[0138] The third step: use the verification set to verify the recognition structure separately, and verify the result of decision fusion;
[0139] Step 4: Use the test set to test the entire algorithm. If the test result meets the requirements, it ends. Otherwise, return to the second step and train again.
[0140] Recognition process: After the video sequence stream to be recognized is regularized into a video sequence stream of a specified length, the trained deep learning network architecture is input for recognition, and the classi...
PUM
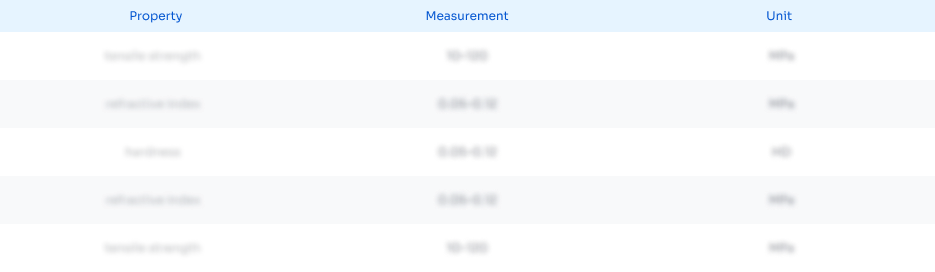
Abstract
Description
Claims
Application Information
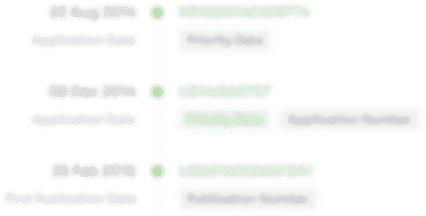
- R&D Engineer
- R&D Manager
- IP Professional
- Industry Leading Data Capabilities
- Powerful AI technology
- Patent DNA Extraction
Browse by: Latest US Patents, China's latest patents, Technical Efficacy Thesaurus, Application Domain, Technology Topic, Popular Technical Reports.
© 2024 PatSnap. All rights reserved.Legal|Privacy policy|Modern Slavery Act Transparency Statement|Sitemap|About US| Contact US: help@patsnap.com