Video super-resolution method based on convolutional neural network and mixed resolution
A convolutional neural network and super-resolution technology, applied in the field of video super-resolution based on convolutional neural network and mixed resolution, can solve the problem of unsatisfactory super-resolution results, lack of high-frequency information including texture details, increased Problems such as the overall calculation redundancy of video sequences, to achieve the effect of increasing nonlinear mapping capabilities, improving processing capabilities, and improving recovery capabilities
- Summary
- Abstract
- Description
- Claims
- Application Information
AI Technical Summary
Problems solved by technology
Method used
Image
Examples
Embodiment Construction
[0032] The present invention will be further described in detail below in conjunction with the accompanying drawings and specific embodiments.
[0033] This embodiment provides a video super-resolution method based on a convolutional neural network and a mixed resolution model, and the specific steps are as follows:
[0034] Step 1, data preprocessing stage: collect Internet video sequences to form a data set, which contains sports, natural scenery, animal migration, building movement and other different scenes; in this embodiment, some video sequences in the data set are not compressed at all The video with a resolution of 3840×2160 is down-sampled by 4 times to convert the resolution to 940×540; the resolution of other videos is around 1080×720;
[0035] Step 2, data set division: all the video sequences in the data set are randomly sampled; in this embodiment, 70 scenes are selected as the training data set, of which 64 scenes are used for the training of the network model,...
PUM
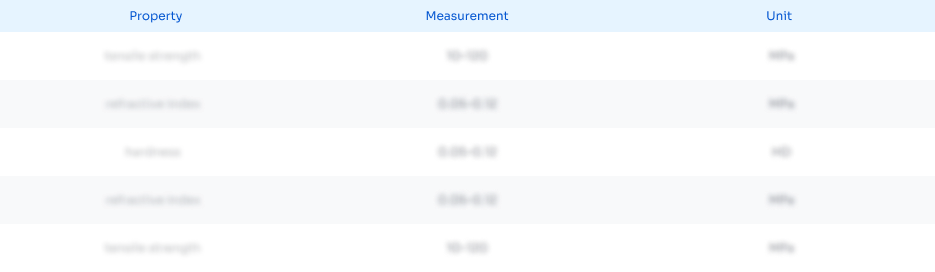
Abstract
Description
Claims
Application Information
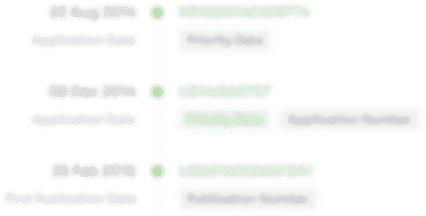
- R&D
- Intellectual Property
- Life Sciences
- Materials
- Tech Scout
- Unparalleled Data Quality
- Higher Quality Content
- 60% Fewer Hallucinations
Browse by: Latest US Patents, China's latest patents, Technical Efficacy Thesaurus, Application Domain, Technology Topic, Popular Technical Reports.
© 2025 PatSnap. All rights reserved.Legal|Privacy policy|Modern Slavery Act Transparency Statement|Sitemap|About US| Contact US: help@patsnap.com