Low-frequency magnetotelluric signal denoising method based on shift invariant sparse coding
A sparse coding, magnetotelluric technology, applied in the field of exploration geophysical signal processing, can solve problems such as difficulty in data processing, loss of low-frequency effective signals in strong interference segments, and few methods for signal-to-noise separation
- Summary
- Abstract
- Description
- Claims
- Application Information
AI Technical Summary
Problems solved by technology
Method used
Image
Examples
Embodiment 1
[0067] Applying the signal-to-noise separation method described in the present invention to the processing of synthetic data, it is obtained as figure 2 The experimental results shown. figure 2 Middle (a) is a time series segment collected in the Qaidam Basin in Qinghai, with a sampling frequency of 15 Hz. Obviously, there is no obvious strong human noise in this time series, and the signal changes smoothly, which is high-quality data; figure 2 (b) is the noisy signal obtained after adding the simulated strong humanistic noise in (a), figure 2 (c) is the result of denoising directly using the mathematical morphological filtering method. It is not difficult to find that the morphological filtering method removes almost all low-frequency slow-changing parts while denoising, and loses a large amount of low-frequency effective signals, and there are still a small amount of Impulse noise; figure 2 (d) is the signal obtained after using the method described in the present inv...
Embodiment 2
[0069] Similar to Example 1, the signal-to-noise separation method of the present invention is applied to the processing of the measured time series in Qinghai, and the following results are obtained: image 3 The experimental results shown. It is not difficult to find that before denoising image 3 (a), the time series contains a large amount of impulse noise, and its amplitude is significantly larger than the effective signal. After denoising image 3 (b), the impulse noise is suppressed, and the low-frequency slowly changing signal is well preserved.
Embodiment 3
[0071] Similar to Embodiment 1 and Embodiment 2, the signal-to-noise separation method of the present invention is applied to the measured magnetotelluric data of the Tongling ore concentration area in Anhui. get as Figure 4 The experimental results shown. Such as Figure 4 As shown in (a), the pre-processing time series contains a lot of pulse and square wave noise, and its amplitude is significantly larger than the effective signal. Such as Figure 4 As shown in (b), after using the present invention for denoising, square wave noise and impulse noise are accurately removed. Likewise, there is no noticeable loss of low frequency signals.
[0072] The beneficial effects of the present invention are illustrated by comparing the apparent resistivity-phase curves before and after processing using the signal-to-noise separation method of the present invention. Such as Figure 5 Shown are the apparent resistivity-phase curves before and after processing of a measured point i...
PUM
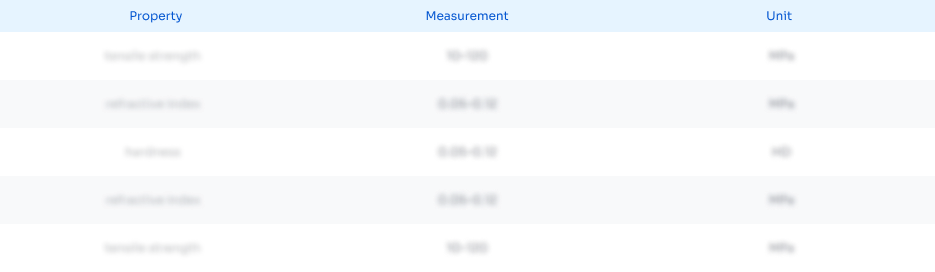
Abstract
Description
Claims
Application Information
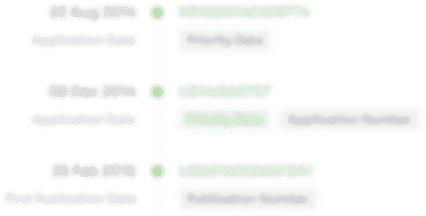
- R&D Engineer
- R&D Manager
- IP Professional
- Industry Leading Data Capabilities
- Powerful AI technology
- Patent DNA Extraction
Browse by: Latest US Patents, China's latest patents, Technical Efficacy Thesaurus, Application Domain, Technology Topic, Popular Technical Reports.
© 2024 PatSnap. All rights reserved.Legal|Privacy policy|Modern Slavery Act Transparency Statement|Sitemap|About US| Contact US: help@patsnap.com