Power point commodity recommendation method and system based on logistic regression
A product recommendation and logistic regression technology, which is applied in the direction of business, equipment, sales/lease transactions, etc., can solve the problems of weak recommendation targets, increased system burden, and no consideration of user behavior habits, etc., to meet the real-time requirements of the system Effect
- Summary
- Abstract
- Description
- Claims
- Application Information
AI Technical Summary
Problems solved by technology
Method used
Image
Examples
Embodiment 1
[0038] Embodiment 1, this embodiment provides a method for recommending power points commodities based on logistic regression;
[0039] A method for recommending power points products based on logistic regression, including:
[0040] S0: Cluster users to obtain different user groups;
[0041] S1: For each type of user, obtain the most relevant feature data of positive sample users who have points and have redeemed products with points, and also obtain the most relevant feature data of negative sample users who have points and have not redeemed products with points;
[0042] S2: Using the logistic regression algorithm, the most relevant feature data of positive sample users, the most relevant feature data of negative sample users, and the label of points redemption or not are used as training set data to establish a potential customer prediction model;
[0043] S3: Based on the potential customer prediction model, predict the probability of the user's points exchange according...
Embodiment 2
[0098] Embodiment 2: This embodiment provides a power point commodity recommendation system based on logistic regression;
[0099] A power point commodity recommendation system based on logistic regression, including:
[0100] User classification module, which clusters users to obtain different user groups;
[0101] The most relevant feature acquisition module, which is configured to obtain the most relevant feature data of positive sample users who have points and have redeemed commodities with points for each type of user, and also obtain negative samples that have points and have never redeemed commodities with points User's most relevant characteristic data;
[0102] A potential customer prediction model building module, which is configured to adopt a logistic regression algorithm, using the most relevant feature data of positive sample users, the most relevant feature data of negative sample users, and the label of points redemption or not as training set data to establi...
Embodiment 3
[0105] Embodiment 3: This embodiment also provides an electronic device, including a memory, a processor, and computer instructions stored in the memory and run on the processor. When the computer instructions are executed by the processor, each step in the method is completed. For the sake of brevity, the operation will not be repeated here.
[0106] Described electronic device can be mobile terminal and non-mobile terminal, and non-mobile terminal comprises desktop computer, and mobile terminal comprises smart phone (Smart Phone, such as Android mobile phone, IOS mobile phone etc.), smart glasses, smart watch, smart bracelet, tablet computer , laptops, personal digital assistants and other mobile Internet devices that can communicate wirelessly.
PUM
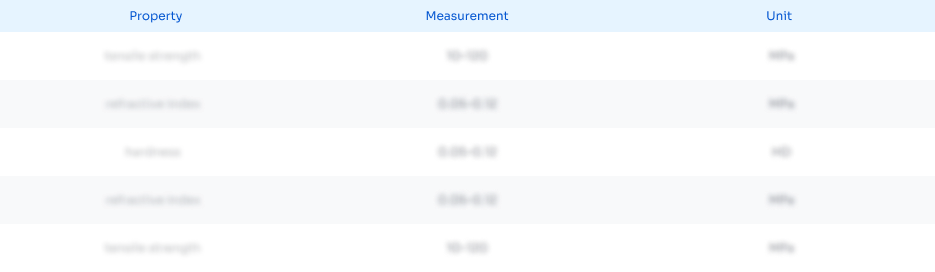
Abstract
Description
Claims
Application Information
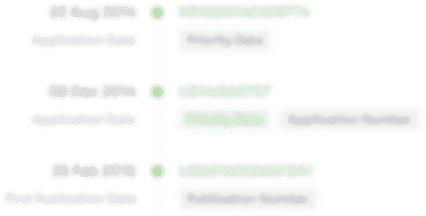
- R&D Engineer
- R&D Manager
- IP Professional
- Industry Leading Data Capabilities
- Powerful AI technology
- Patent DNA Extraction
Browse by: Latest US Patents, China's latest patents, Technical Efficacy Thesaurus, Application Domain, Technology Topic, Popular Technical Reports.
© 2024 PatSnap. All rights reserved.Legal|Privacy policy|Modern Slavery Act Transparency Statement|Sitemap|About US| Contact US: help@patsnap.com