Low elevation angle DOA estimation method based on RBF neural network
A neural network, low-elevation technology, applied in the field of radar, can solve the problems of inability to effectively separate source coherent signals, inability to estimate the direction of arrival, slow DOA estimation, etc. The effect of improved angular accuracy
- Summary
- Abstract
- Description
- Claims
- Application Information
AI Technical Summary
Problems solved by technology
Method used
Image
Examples
Embodiment 1
[0041] See figure 1 , figure 1 It is a flowchart of a low-elevation DOA estimation method based on an RBF neural network provided by an embodiment of the present invention;
[0042] A kind of low-elevation DOA estimation method based on RBF neural network provided by the present invention comprises the following steps:
[0043] S1: Select the traces whose elevation angle is low in the measured data, and use the real elevation angle corresponding to the traces of the low elevation angle as the label Y of the training neural network, Y=[y 1 ,y 2 ,...,y n ], according to the label y i Get the corresponding data covariance matrix R i , from the data covariance matrix R i Extract the corresponding real part features and imaginary part features to get the column vector r i ;
[0044] In this embodiment, assuming that the receiving array is a uniform linear array of M array elements, the array receiving signal X(t) is: X(t)=AS(t)+N(t), where X(t) =[x 1 (t),x 2 (t),...,x M...
Embodiment 2
[0062] In order to make the technical problems, technical solutions and advantages to be solved by the present invention clearer, the following will describe in detail on the basis of the above-mentioned embodiments with reference to the accompanying drawings and specific experiments.
[0063] In this embodiment, the experimental conditions are as follows:
[0064] The target elevation angles used for testing and training are all low elevation angles below 5°, and 25 tracks in the azimuth sector under the complex position within 180°-210° and 330°-360° are selected for analysis, 18 of which are For the training of the RBF network, the training set has a total of 2661 traces, 7 tracks are used for the test of the RBF network, and the test set has a total of 892 traces. The data processing and neural network training part of the experiment were completed on MATLAB2017a. For the training track diagram and test track diagram, please refer to image 3 , image 3 It is the traini...
PUM
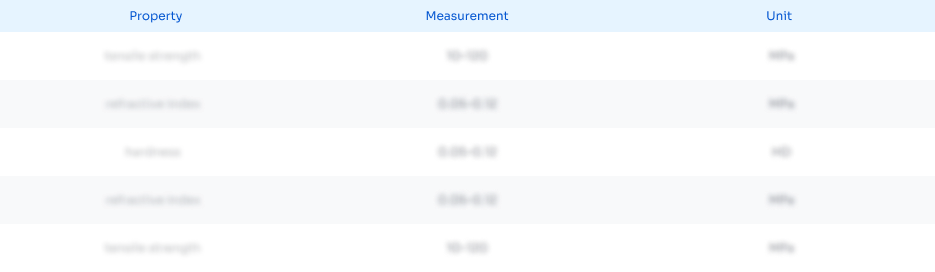
Abstract
Description
Claims
Application Information
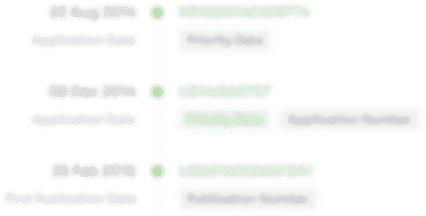
- R&D Engineer
- R&D Manager
- IP Professional
- Industry Leading Data Capabilities
- Powerful AI technology
- Patent DNA Extraction
Browse by: Latest US Patents, China's latest patents, Technical Efficacy Thesaurus, Application Domain, Technology Topic, Popular Technical Reports.
© 2024 PatSnap. All rights reserved.Legal|Privacy policy|Modern Slavery Act Transparency Statement|Sitemap|About US| Contact US: help@patsnap.com