Abnormal behavior detection method based on improved pseudo three-dimensional residual neural network
A neural network and detection method technology, applied in the field of video analysis, can solve problems such as blurred boundaries, complex calculations, high labor costs, etc., and achieve the effect of improving accuracy
- Summary
- Abstract
- Description
- Claims
- Application Information
AI Technical Summary
Problems solved by technology
Method used
Image
Examples
Embodiment Construction
[0040] The purpose of the present invention is to provide an abnormal behavior detection method in a monitoring video scene, specifically an abnormal behavior detection method based on multi-instance learning and an improved pseudo three-dimensional residual neural network, so as to strengthen monitoring capabilities and improve public safety.
[0041] Such as figure 1 Shown, the implementation steps of the present invention are as follows:
[0042] First, divide each video in the training set into multiple video clips, and input the improved P3D-ResNet to get their features; then, take the feature average of all frames in each video clip, and then perform the feature average The L2 norm is normalized to obtain the features of the video; finally, these features are input into a 3-layer fully connected neural network, which will output the anomaly score of the video clip.
[0043] The three pseudo three-dimensional residual block structures in the residual network constructed ...
PUM
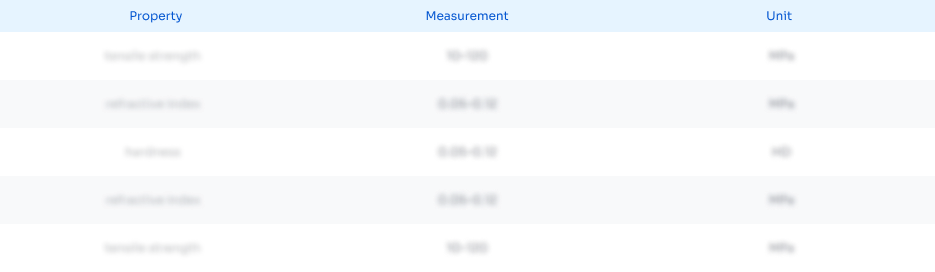
Abstract
Description
Claims
Application Information
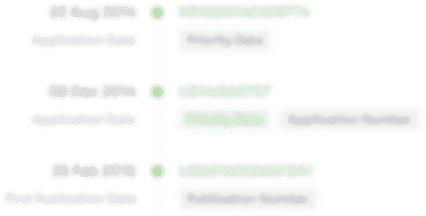
- R&D Engineer
- R&D Manager
- IP Professional
- Industry Leading Data Capabilities
- Powerful AI technology
- Patent DNA Extraction
Browse by: Latest US Patents, China's latest patents, Technical Efficacy Thesaurus, Application Domain, Technology Topic, Popular Technical Reports.
© 2024 PatSnap. All rights reserved.Legal|Privacy policy|Modern Slavery Act Transparency Statement|Sitemap|About US| Contact US: help@patsnap.com