Wind turbine generator fault prediction method based on deep learning
A technology of fault prediction and wind turbines, applied in the direction of neural learning methods, prediction, computer components, etc., can solve the problem of fault data feature learning ability, long-term high real-time state data processing ability, prediction efficiency and accuracy limitations, massive time-series data Effectively deal with issues such as means to achieve the effect of improving the time span
- Summary
- Abstract
- Description
- Claims
- Application Information
AI Technical Summary
Problems solved by technology
Method used
Image
Examples
Embodiment Construction
[0058] The present invention will be further described below in conjunction with the accompanying drawings. The following examples are only used to illustrate the technical solution of the present invention more clearly, but not to limit the protection scope of the present invention.
[0059] A fault prediction method for wind turbines based on deep learning of the present invention, the fault prediction method is aimed at the SCADA data of the operating state of the wind turbines, and the fault prediction method performs fault prediction based on a fault prediction model;
[0060] Such as figure 1 As shown, the fault prediction model includes a feature extraction module, a fault prediction module and a fully connected layer;
[0061] The feature extraction module is constructed by a multi-layer convolutional neural network structure (i.e. a deep convolutional neural network), and is used to extract fault features of multi-frame operating data graphs;
[0062] In the embodim...
PUM
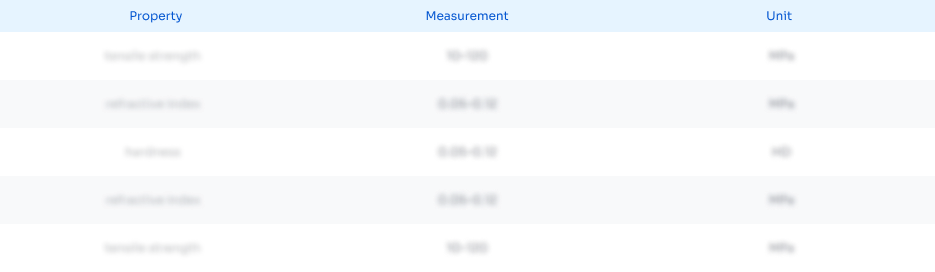
Abstract
Description
Claims
Application Information
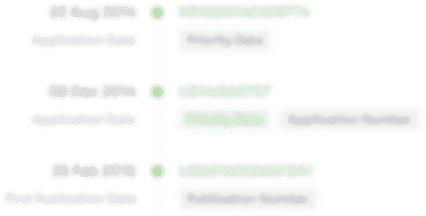
- R&D Engineer
- R&D Manager
- IP Professional
- Industry Leading Data Capabilities
- Powerful AI technology
- Patent DNA Extraction
Browse by: Latest US Patents, China's latest patents, Technical Efficacy Thesaurus, Application Domain, Technology Topic.
© 2024 PatSnap. All rights reserved.Legal|Privacy policy|Modern Slavery Act Transparency Statement|Sitemap