Construction method of fabric defect recognition system based on lightweight convolutional neural network
A convolutional neural network and defect recognition technology, applied in the field of textile image recognition, can solve problems such as large amount of calculation and complex structure, and achieve the effect of easy operation and reduced dependence on hardware computing power and memory capacity
- Summary
- Abstract
- Description
- Claims
- Application Information
AI Technical Summary
Problems solved by technology
Method used
Image
Examples
Embodiment Construction
[0033] The technical solutions in the embodiments of the present invention will be clearly and completely described below in conjunction with the accompanying drawings in the embodiments of the present invention. Obviously, the described embodiments are only a part of the embodiments of the present invention, rather than all the embodiments. Based on the embodiments of the present invention, all other embodiments obtained by those of ordinary skill in the art without creative work shall fall within the protection scope of the present invention.
[0034] Such as figure 1 As shown, a method for building a fabric defect recognition system based on a lightweight convolutional neural network, the steps are as follows:
[0035] S1. Configure the operating environment of the fabric defect recognition system; the operating environment of the fabric defect recognition system includes a hardware system and a software system. The processor of the hardware system includes two CPUs and two GPUs...
PUM
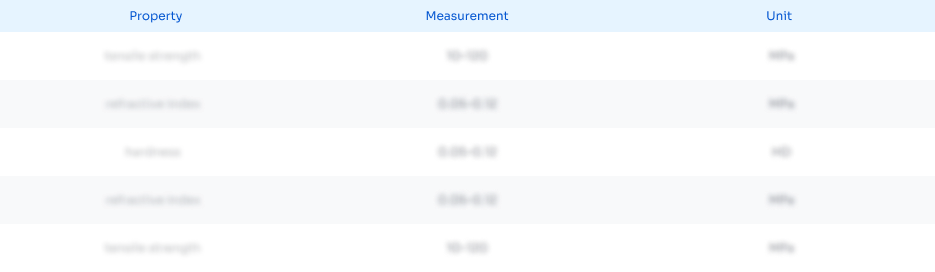
Abstract
Description
Claims
Application Information
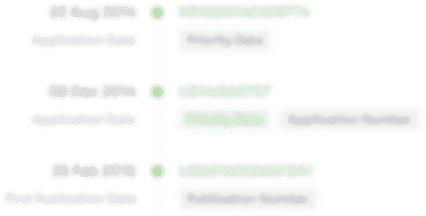
- R&D Engineer
- R&D Manager
- IP Professional
- Industry Leading Data Capabilities
- Powerful AI technology
- Patent DNA Extraction
Browse by: Latest US Patents, China's latest patents, Technical Efficacy Thesaurus, Application Domain, Technology Topic, Popular Technical Reports.
© 2024 PatSnap. All rights reserved.Legal|Privacy policy|Modern Slavery Act Transparency Statement|Sitemap|About US| Contact US: help@patsnap.com