Federated deep learning method capable of adaptively protecting privacy
A privacy protection and deep learning technology, applied in machine learning, digital data protection, instruments, etc., to achieve the effect of increasing accuracy, increasing privacy, and improving accuracy
- Summary
- Abstract
- Description
- Claims
- Application Information
AI Technical Summary
Problems solved by technology
Method used
Image
Examples
Embodiment Construction
[0025] 1. The system model of the invention is as figure 1 Shown:
[0026] 2. System initialization, including the following steps:
[0027] 1) Participant U g A learning model that is more accurate and does not lead to local overfitting needs to be jointly obtained, and the participant U g Negotiate a deep learning network with the cloud server in advance, such as convolutional neural network CNN, recurrent neural network RNN, etc.;
[0028] 2) The server uses some public data for training according to the user data type, and obtains an initialized deep learning model parameter w global ;
[0029] 3. The participant initializes the local model, which is characterized in that it includes the following steps:
[0030] 1) The server broadcasts its own deep learning model parameter w global ;
[0031] 2) Each participant U g Download the initialized model parameters w global , and update its own local learning model w local ;
[0032] 4. The participant preprocesses th...
PUM
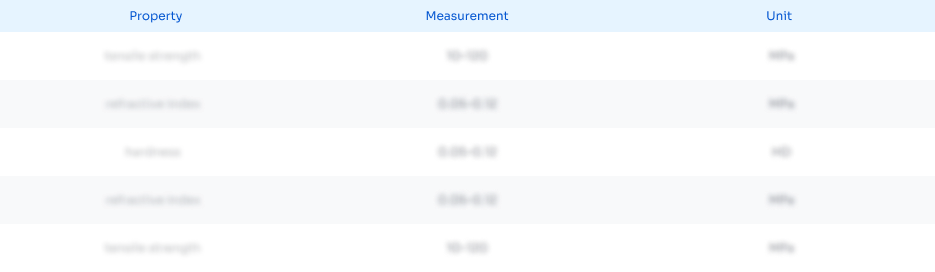
Abstract
Description
Claims
Application Information
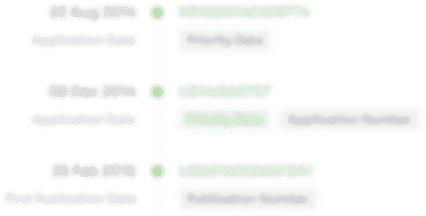
- Generate Ideas
- Intellectual Property
- Life Sciences
- Materials
- Tech Scout
- Unparalleled Data Quality
- Higher Quality Content
- 60% Fewer Hallucinations
Browse by: Latest US Patents, China's latest patents, Technical Efficacy Thesaurus, Application Domain, Technology Topic, Popular Technical Reports.
© 2025 PatSnap. All rights reserved.Legal|Privacy policy|Modern Slavery Act Transparency Statement|Sitemap|About US| Contact US: help@patsnap.com