A ddnn based on cloud-edge collaborative computing and its construction method and application
An edge and edge-side technology, applied to DDNN based on cloud-edge collaborative computing and its construction field, can solve the problem of low overall performance of DDNN, achieve the effect of improving accuracy, improving classification accuracy, and increasing effectiveness
- Summary
- Abstract
- Description
- Claims
- Application Information
AI Technical Summary
Problems solved by technology
Method used
Image
Examples
Embodiment 1
[0060] A DDNN based on cloud-edge collaborative computing is applied to object classification under multi-view images of the Internet of Things, including edge side and cloud.
[0061] Among them, the edge side is used to extract the features of each viewing angle image, and the feature bag model is used to measure the similarity of the extracted feature images and obtain the histogram vector of the viewing angle; the histogram vector of each viewing angle Based on the fused histogram vector, the target classification on the edge side is obtained. If the target classification does not meet the accuracy requirements, multiple feature images of each view are transmitted to the cloud; the cloud is used for all Feature weighted fusion is performed between multiple feature images, and convolution and classification operations are performed on the fused feature images to obtain the target classification in the cloud.
[0062] This embodiment introduces a bag-of-features model on the...
Embodiment 2
[0089] A method 100 for constructing a DDNN based on cloud-edge collaborative computing as described in Embodiment 1 above, including:
[0090] Step 110, establishing the edge side regression model and the cloud regression model of DDNN respectively;
[0091] Step 120, based on the edge side regression model and the cloud regression model, according to the actual needs of classification accuracy, communication volume and communication time, determine the edge side parameters and cloud parameters, and obtain DDNN.
[0092] This embodiment provides a method for constructing DDNN. Specifically, the regression model of DDNN on the edge side and the regression model of cloud DDNN are introduced first. The actual limit of the amount and the actual limit of the communication time are beneficial to the regression model, obtaining the parameters of the edge side and the cloud, and then quickly and efficiently constructing the DDNN that is actually required. This construction method ca...
Embodiment 3
[0108] A target classification method under multi-view images of the Internet of Things, comprising:
[0109] Collect multi-view images of the target;
[0110] Based on the multi-view image, use any DDNN based on cloud-edge collaborative computing as described in the first embodiment above to obtain the target classification; or determine the edge side and the cloud based on the DDNN construction method described in the second embodiment above to obtain target classification.
[0111] Using the above-mentioned DDNN can reduce the resource occupation of the edge side and improve the classification accuracy. The relevant technical solutions are the same as those in Embodiment 1, and will not be repeated here.
PUM
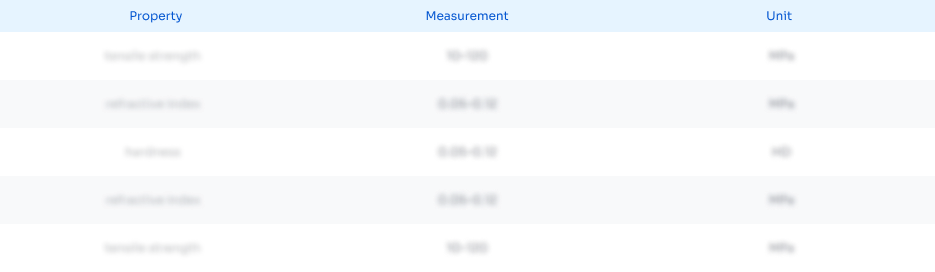
Abstract
Description
Claims
Application Information
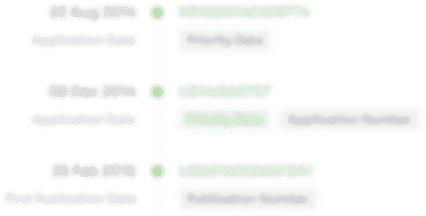
- R&D Engineer
- R&D Manager
- IP Professional
- Industry Leading Data Capabilities
- Powerful AI technology
- Patent DNA Extraction
Browse by: Latest US Patents, China's latest patents, Technical Efficacy Thesaurus, Application Domain, Technology Topic, Popular Technical Reports.
© 2024 PatSnap. All rights reserved.Legal|Privacy policy|Modern Slavery Act Transparency Statement|Sitemap|About US| Contact US: help@patsnap.com