Enterprise power consumption maximum demand prediction method based on ARIMA and SVM
A forecasting method and technology of electricity consumption, applied in forecasting, nuclear methods, data processing applications, etc., can solve the problems of forecast deviation of future monthly maximum demand, lack of enterprise electricity load characteristics, and insufficient enterprise users.
- Summary
- Abstract
- Description
- Claims
- Application Information
AI Technical Summary
Problems solved by technology
Method used
Image
Examples
Embodiment 1
[0099] Such as figure 1 Shown, a kind of future monthly maximum demand prediction method based on differential integrated moving average autoregressive model (ARIMA) time series prediction and support vector machine (SVM), described method implementation process is as follows:
[0100] 1. To preprocess the data, first fill in the missing values in the uploaded data, because the uploaded meter reading value records the total power displayed by the meter every 15 minutes, and the calculation of the time period has to be done poorly, and sometimes "0" will be uploaded value, resulting in data errors, we use the moving average method to fill in missing values;
[0101] 2. If Figure 5 As shown, the K-Means clustering method is used to remove outliers to make the curve converge better. The specific calculation process is as follows:
[0102] 1) Randomly select K center points;
[0103] 2) Assign each data point to its nearest center point;
[0104] 3) Recalculate the average...
PUM
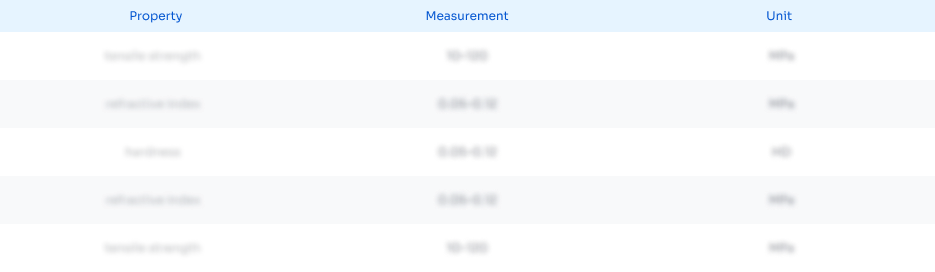
Abstract
Description
Claims
Application Information
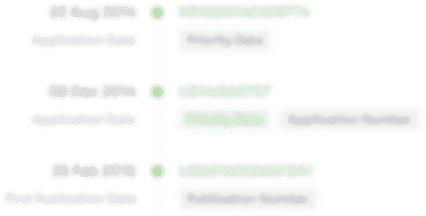
- R&D Engineer
- R&D Manager
- IP Professional
- Industry Leading Data Capabilities
- Powerful AI technology
- Patent DNA Extraction
Browse by: Latest US Patents, China's latest patents, Technical Efficacy Thesaurus, Application Domain, Technology Topic, Popular Technical Reports.
© 2024 PatSnap. All rights reserved.Legal|Privacy policy|Modern Slavery Act Transparency Statement|Sitemap|About US| Contact US: help@patsnap.com