Commodity recommendation method and system based on user session and graph convolutional neural network
A convolutional neural network and product recommendation technology, applied in the field of user preference product recommendation, can solve problems such as long time, many training parameters, and reduced product recommendation efficiency, and achieve the effect of improving accuracy and speed, and improving accuracy
- Summary
- Abstract
- Description
- Claims
- Application Information
AI Technical Summary
Problems solved by technology
Method used
Image
Examples
Embodiment 1
[0029] figure 1 The flow chart of the product recommendation method based on user session and graph convolutional neural network in this embodiment is given.
[0030] to combine figure 1 , the product recommendation method based on user sessions and graph convolutional neural networks in this embodiment includes:
[0031] S101: Receive the clicked commodity sequence in the same time unit, model it as a session in the form of a directed graph, and construct a session graph; wherein, the session graph is expressed in the form of an embedding vector.
[0032] In step S101, the specific process of constructing the session graph is as follows:
[0033] Obtain a click sequence of the user within the same time unit, forming a session. For example, if the click sequence generated by a user on a shopping website on July 21 is (dress→high heels→necklace→skirt), then such a click sequence becomes a session.
[0034] Use the divided session to model in the form of a directed graph, su...
Embodiment 2
[0114] figure 2 A schematic structural diagram of the product recommendation system based on user sessions and graph convolutional neural networks in this embodiment is given.
[0115] combine figure 2 , the product recommendation system based on user sessions and graph convolutional neural networks in this embodiment includes:
[0116] (1) Conversation graph building module, which is used to receive the clicked product sequence in the same time unit, as a session and modeled in the form of a directed graph, to construct a session graph; wherein, the session graph is expressed in the form of an embedded vector ;
[0117] Specifically, in the session graph construction module, each node in the session graph represents a commodity, each edge is the order in which the commodities are clicked, and the weight of each edge is equal to the number of occurrences of the edge divided by the starting point of the edge. The out-degree of the starting node; each product is embedded in...
Embodiment 3
[0141] This embodiment provides a computer-readable storage medium on which a computer program is stored, and when the program is executed by a processor, the steps in the product recommendation method based on user session and graph convolutional neural network as described in Embodiment 1 are realized. .
[0142]In this embodiment, by constructing a session graph, comprehensively paying attention to the user's long-term and short-term preferences and fluctuating discrete preferences, considering the internal order of each session of the user and the correlation between multiple sessions, it is possible to consider complex data forms and Network structure, input the conversation graph into the gated graph neural network, output the posterior probabilities of all commodities contained in the conversation graph, use the magnitude of the posterior probability to output commodity recommendation results, and improve the The accuracy and speed of product recommendation are improved...
PUM
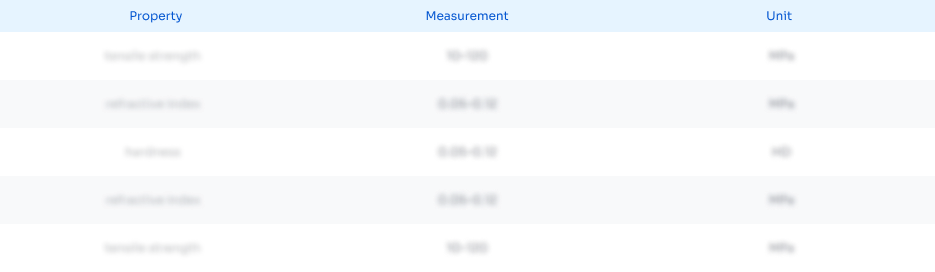
Abstract
Description
Claims
Application Information
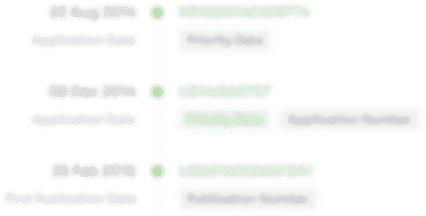
- R&D Engineer
- R&D Manager
- IP Professional
- Industry Leading Data Capabilities
- Powerful AI technology
- Patent DNA Extraction
Browse by: Latest US Patents, China's latest patents, Technical Efficacy Thesaurus, Application Domain, Technology Topic, Popular Technical Reports.
© 2024 PatSnap. All rights reserved.Legal|Privacy policy|Modern Slavery Act Transparency Statement|Sitemap|About US| Contact US: help@patsnap.com