Camera attitude estimation method based on deep neural network
A technology of deep neural network and camera pose, which is applied in the field of camera pose estimation based on deep neural network, can solve the problem of poor performance of pose network and achieve the effect of improving performance
- Summary
- Abstract
- Description
- Claims
- Application Information
AI Technical Summary
Problems solved by technology
Method used
Image
Examples
Embodiment Construction
[0028] The present invention will be further described in detail below in conjunction with the accompanying drawings and through specific embodiments. The following embodiments are only descriptive, not restrictive, and cannot limit the protection scope of the present invention.
[0029] The camera pose estimation method based on the deep neural network of the present invention adopts an unsupervised training method, and introduces a joint training strategy of optical flow and pose, so that the extracted features have scene geometric features and improve the accuracy of pose estimation.
[0030] Specific steps are as follows:
[0031] 1) Build a camera pose estimation network, such as figure 1 As shown, the model is designed based on a stacked convolutional neural network structure, including convolutional layers, deconvolutional layers, and fully connected layers;
[0032] The pose estimation network of the present invention is mainly composed of three sub-networks, includin...
PUM
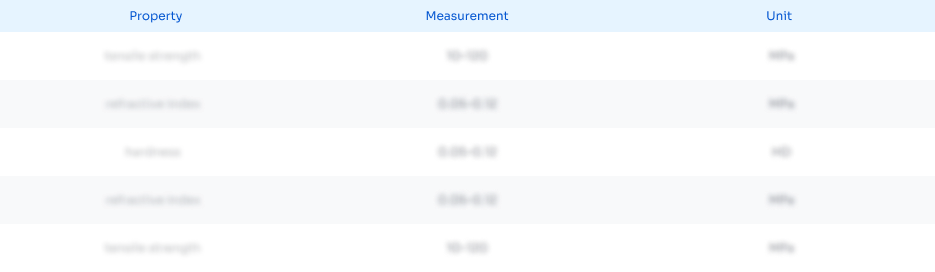
Abstract
Description
Claims
Application Information
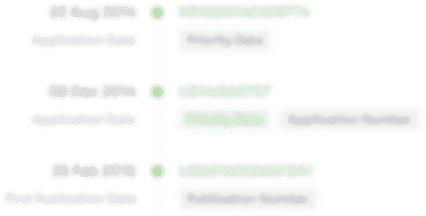
- R&D
- Intellectual Property
- Life Sciences
- Materials
- Tech Scout
- Unparalleled Data Quality
- Higher Quality Content
- 60% Fewer Hallucinations
Browse by: Latest US Patents, China's latest patents, Technical Efficacy Thesaurus, Application Domain, Technology Topic, Popular Technical Reports.
© 2025 PatSnap. All rights reserved.Legal|Privacy policy|Modern Slavery Act Transparency Statement|Sitemap|About US| Contact US: help@patsnap.com