Method for establishing word vector improved model based on semantic embedding
A technology for improving models and building methods, applied in the field of word vector model building, can solve problems such as difficult to distinguish the meaning of polysemous words
- Summary
- Abstract
- Description
- Claims
- Application Information
AI Technical Summary
Problems solved by technology
Method used
Image
Examples
Embodiment Construction
[0070] Below in conjunction with accompanying drawing and specific embodiment, further illustrate the present invention, should be understood that these examples are only for illustrating the present invention and are not intended to limit the scope of the present invention, after having read the present invention, those skilled in the art will understand various aspects of the present invention All modifications of the valence form fall within the scope defined by the appended claims of the present application.
[0071] A method for building an improved word vector model based on semantic embedding, such as Figure 1-4 As shown, the method mainly includes three stages, namely: context vector training stage, semantic induction stage and semantic representation stage. Concretely include the following steps:
[0072] 1) Context vector training phase, ( figure 1 Middle 1-3 steps): such as figure 2 , 3 as shown,
[0073] 1)-a Process a large-scale corpus, extract text conten...
PUM
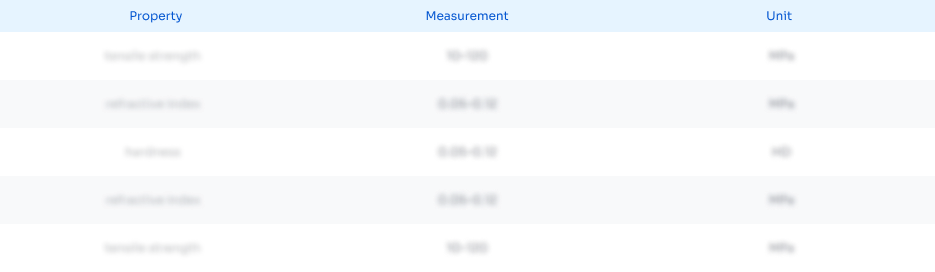
Abstract
Description
Claims
Application Information
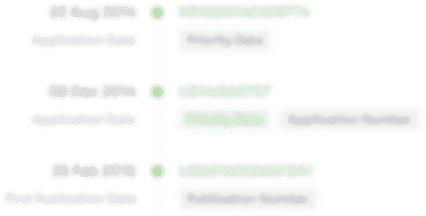
- R&D Engineer
- R&D Manager
- IP Professional
- Industry Leading Data Capabilities
- Powerful AI technology
- Patent DNA Extraction
Browse by: Latest US Patents, China's latest patents, Technical Efficacy Thesaurus, Application Domain, Technology Topic, Popular Technical Reports.
© 2024 PatSnap. All rights reserved.Legal|Privacy policy|Modern Slavery Act Transparency Statement|Sitemap|About US| Contact US: help@patsnap.com