Fault feature learning and classification method based on 1DCNN and GRU fusion
A 1D-CNN, classification method technology, applied in neural learning methods, instruments, biological neural network models, etc., can solve the problem of non-image sequence fault feature extraction and classification, etc.
- Summary
- Abstract
- Description
- Claims
- Application Information
AI Technical Summary
Problems solved by technology
Method used
Image
Examples
Embodiment Construction
[0030] In order to make the purpose, technical solution and advantages of the present invention clearer, the present invention will be further described in detail below in conjunction with specific embodiments of the present invention and with reference to the accompanying drawings. The described embodiments are some, but not all, embodiments of the invention.
[0031] In order to solve some of the deficiencies in the prior art, the embodiments of the present invention provide a fusion fault feature learning and classification method, and apply it to chiller fault feature learning and classification. The feature learning and classification method includes the following step:
[0032] Step 101: Obtain equipment operation data set X∈R d×n , where d represents the dimension of the collected data, and n represents the number of samples; the data used in this example comes from the 1043 research project initiated by the American Society of Heating, Refrigerating and Air-Conditioni...
PUM
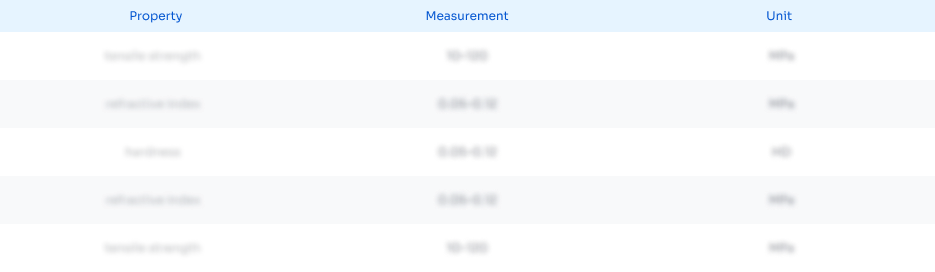
Abstract
Description
Claims
Application Information
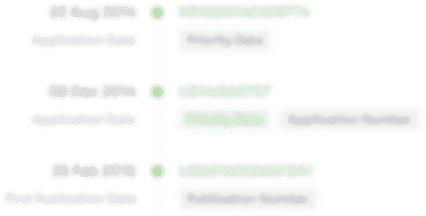
- R&D
- Intellectual Property
- Life Sciences
- Materials
- Tech Scout
- Unparalleled Data Quality
- Higher Quality Content
- 60% Fewer Hallucinations
Browse by: Latest US Patents, China's latest patents, Technical Efficacy Thesaurus, Application Domain, Technology Topic, Popular Technical Reports.
© 2025 PatSnap. All rights reserved.Legal|Privacy policy|Modern Slavery Act Transparency Statement|Sitemap|About US| Contact US: help@patsnap.com