Power load prediction method and system based on deep belief network
A deep belief network and power load technology, applied in forecasting, neural learning methods, biological neural network models, etc., can solve problems that are difficult to deal with the challenges of multi-dimensional data forecasting accuracy, and achieve improved forecasting accuracy, efficiency, and forecasting accuracy Effect
- Summary
- Abstract
- Description
- Claims
- Application Information
AI Technical Summary
Problems solved by technology
Method used
Image
Examples
Embodiment Construction
[0025] In order to further understand the invention content, characteristics and effects of the present invention, the following embodiments are enumerated hereby, and detailed descriptions are as follows in conjunction with the accompanying drawings:
[0026] The Chinese meanings of English abbreviations in this application are as follows:
[0027] DBN: Deep Belief Network prediction model;
[0028] RBM: Restricted Boltzmann Machine;
[0029] GB-RBM: Gauss-Bernoulli Restricted Boltzmann Machine;
[0030] BB-RBM: Bernoulli-Bernoulli Restricted Boltzmann Machine;
[0031] AE: self-encoding neural network;
[0032] SAE: Sparse Autoencoder Neural Network;
[0033] SVM: support vector machine;
[0034] BP: backpropagation algorithm;
[0035] S-BP: standard error backpropagation algorithm based on gradient descent;
[0036] I-BP: An error backpropagation algorithm that adds an impulse item to the weight update rule;
[0037] Gibbs: Gibbs sampling;
[0038] MAPE: Mean Absol...
PUM
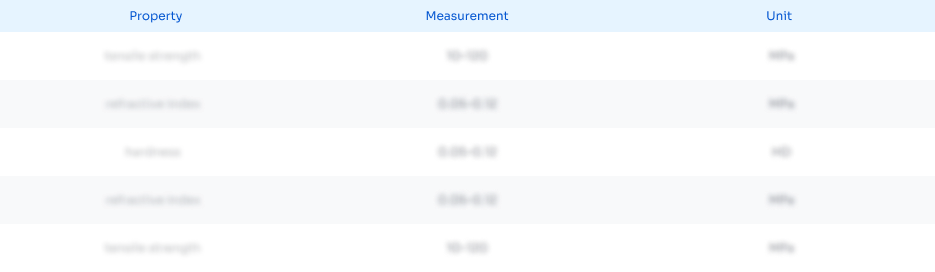
Abstract
Description
Claims
Application Information
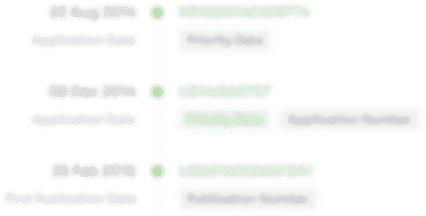
- R&D Engineer
- R&D Manager
- IP Professional
- Industry Leading Data Capabilities
- Powerful AI technology
- Patent DNA Extraction
Browse by: Latest US Patents, China's latest patents, Technical Efficacy Thesaurus, Application Domain, Technology Topic, Popular Technical Reports.
© 2024 PatSnap. All rights reserved.Legal|Privacy policy|Modern Slavery Act Transparency Statement|Sitemap|About US| Contact US: help@patsnap.com