Two-dimensional Inverse-Free Sparse Bayesian Learning for Fast Sparse Reconstruction
A sparse Bayesian, sparse reconstruction technology, applied in the field of signal processing, can solve the problem of low efficiency of two-dimensional signal operation
- Summary
- Abstract
- Description
- Claims
- Application Information
AI Technical Summary
Problems solved by technology
Method used
Image
Examples
Embodiment Construction
[0065] The present invention will be further described below in conjunction with accompanying drawing:
[0066] figure 1 It is the general processing flow of the present invention.
[0067] A two-dimensional inverse-free sparse Bayesian learning fast sparse reconstruction method described in the present invention comprises the following four steps:
[0068] S1: Sparse representation modeling for two-dimensional sparse reconstruction problems;
[0069] S2: Perform statistical modeling on vectorized sparse signal x and vectorized noise n;
[0070] S3: Solve the posterior probability of the vectorized sparse signal x, the reciprocal of the vectorized variance γ and the reciprocal of the noise variance α;
[0071] S4: Updating the matrix form Z of the auxiliary variables.
[0072] Using simulation data to conduct experiments, compare the method of the present invention with the IFSBL method (Duan H, Yang L, Fang J, and Li H. Fast Inverse-Free Sparse Bayesian Learning via Relax...
PUM
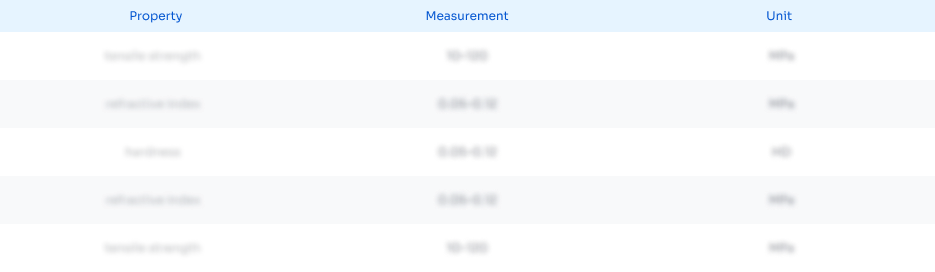
Abstract
Description
Claims
Application Information
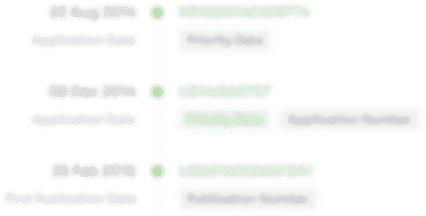
- R&D Engineer
- R&D Manager
- IP Professional
- Industry Leading Data Capabilities
- Powerful AI technology
- Patent DNA Extraction
Browse by: Latest US Patents, China's latest patents, Technical Efficacy Thesaurus, Application Domain, Technology Topic, Popular Technical Reports.
© 2024 PatSnap. All rights reserved.Legal|Privacy policy|Modern Slavery Act Transparency Statement|Sitemap|About US| Contact US: help@patsnap.com