T-closeness privacy protection method satisfying epsilon-differential privacy
A differential privacy and privacy protection technology, applied in digital data protection, instruments, electrical digital data processing, etc., can solve the problem of data loss of meaning, achieve the effect of ensuring the effectiveness of data release, reducing data loss, and protecting relationships
- Summary
- Abstract
- Description
- Claims
- Application Information
AI Technical Summary
Problems solved by technology
Method used
Image
Examples
Embodiment Construction
[0037] The present invention is further described below in conjunction with accompanying drawing. But not limited to the following examples.
[0038] A t-closeness privacy protection method satisfying ε-differential privacy, comprising the following steps:
[0039] Step 1. Preprocess the original data and establish the QIS data table associated with quasi-identifiers and sensitive attributes. The main steps are as follows:
[0040] 1) Establish a correspondence table between quasi-identifier Qi and sensitive attribute Si, each quasi-identifier corresponds to its unique sensitive attribute, as an index table.
[0041] 2) Sort the records using sensitive attributes, and generate different regions by taking k values, iteratively record the k smallest and largest records.
[0042] 3) Store the acquired records in the new data table in turn to generate the final QIS associated data table.
[0043] The algorithm steps are as follows:
[0044] a) Let D={(Qi,Si)|i=1,...,N} be the ...
PUM
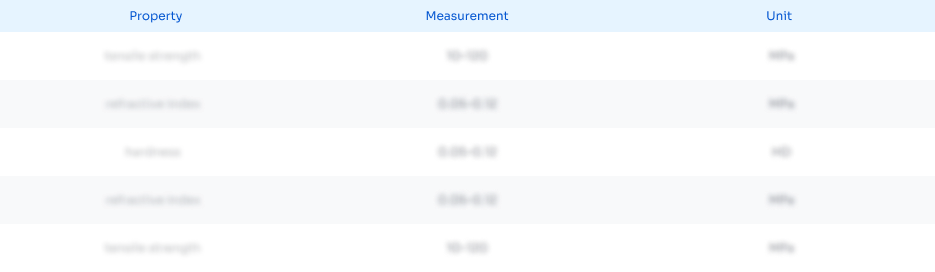
Abstract
Description
Claims
Application Information
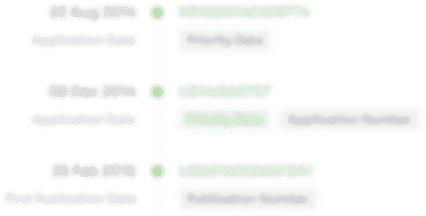
- Generate Ideas
- Intellectual Property
- Life Sciences
- Materials
- Tech Scout
- Unparalleled Data Quality
- Higher Quality Content
- 60% Fewer Hallucinations
Browse by: Latest US Patents, China's latest patents, Technical Efficacy Thesaurus, Application Domain, Technology Topic, Popular Technical Reports.
© 2025 PatSnap. All rights reserved.Legal|Privacy policy|Modern Slavery Act Transparency Statement|Sitemap|About US| Contact US: help@patsnap.com